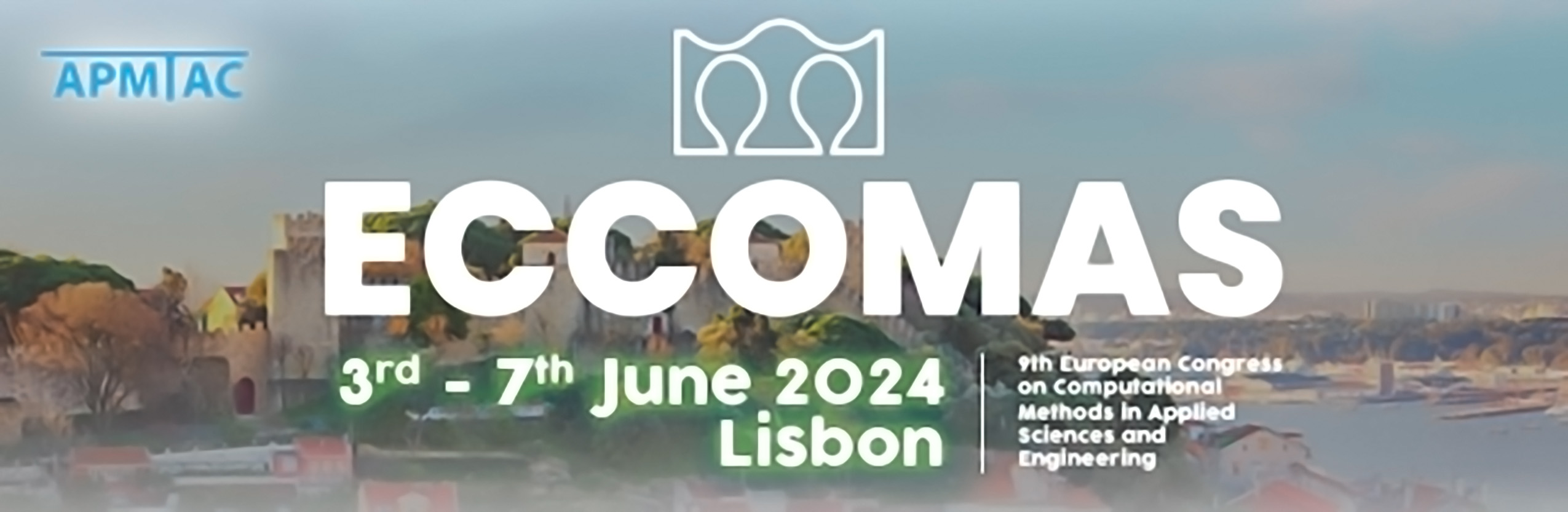
An efficient physics-based model order reduction technique for nonlinear finite element models with finite strains
Please login to view abstract download link
Real-time simulators for minimally invasive surgery are vital for surgeon training, yet simulating soft tissue in real-time with medical devices like imaging catheters poses challenges due to dynamic contact conditions and nonlinear material behaviour. Model Order Reduction (MOR) aims to solve this challenge by solving a reduced set of equations compared to the original Finite Element (FE) model, typically through projection. The changing loading scenarios on soft tissue during the navigation of the imaging catheter make it challenging to train using the data-based MOR approach, Proper Orthogonal Decomposition (POD) [1]. In an alternative physics-based approach [2], the concept of modal derivatives has been used to simulate nonlinear structural dynamics which has since evolved into linear and nonlinear projection-based model order reduction techniques [3], [4]. Nevertheless, these methods have limitations, particularly the lack of orthogonality in the achieved linear projection, which can introduce stability and convergence issues. The Gram-Schmidt process is incorporated in this work to enhance this physics-based approach to ensure an orthogonal projection. This enhanced method Orthogonal Linear Manifold (Orthogonal LM), also involves a greedy selection algorithm that constructs a projection function compromising only dominant modal derivatives and Eigenmodes. Initially, the performance of the Orthogonal LM is compared with POD and Original LM in quasi-statics and finite strain formulation of the complex model. For simple loading scenarios, the Orthogonal LM demonstrates similar performance compared to the Original LM while utilizing fewer basis functions. These physics-based approaches, leveraging their respective greedy selection algorithms, outperform the data-based POD, albeit with only minor differences. However, the Orthogonal LM’s superior performance becomes evident in complex loading scenarios, exhibiting less cumulative displacement error and requiring a reduced number of basis functions to achieve it. Furthermore, Orthogonal LM achieves a computational time reduction of approximately 25 − 30% compared to the original FE model. To further demonstrate the effectiveness of Orthogonal LM, moving load scenarios and problems involving contact with penalty formulation are considered. The results confirm the Orthogonal LM’s superiority over existing MOR techniques.