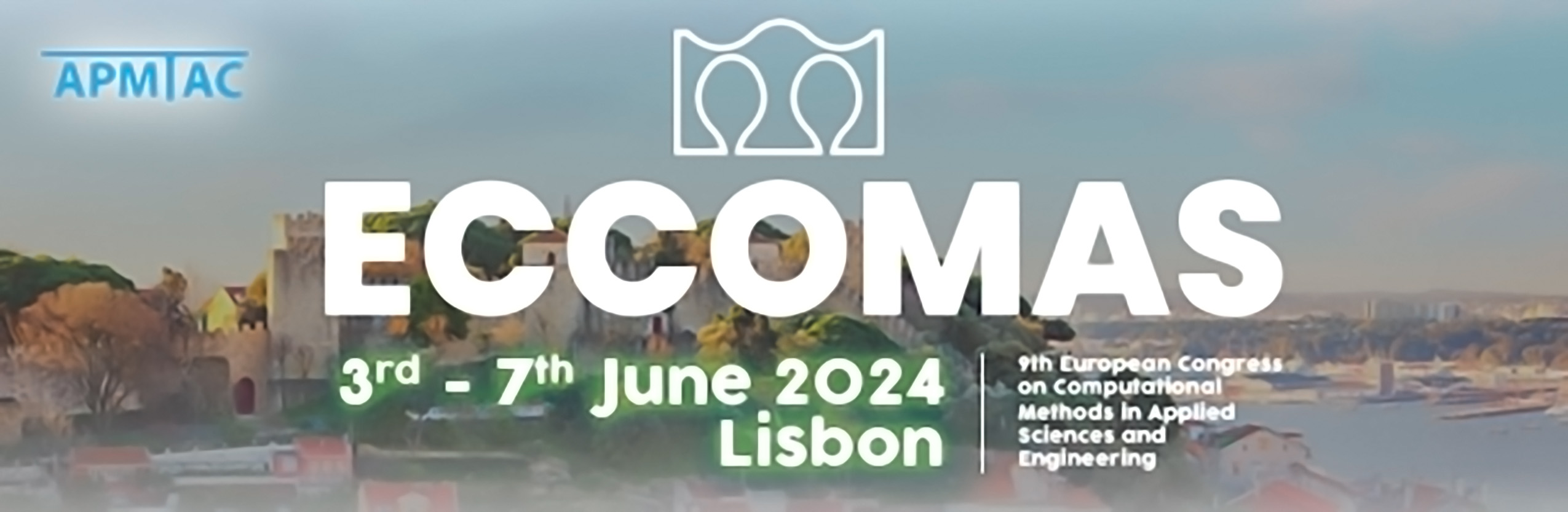
Neural Operator Surrogates for Elliptic PDEs
Please login to view abstract download link
Approximating solution sets of PDEs is an important task for many applications, e.g., model order reduction and uncertainty quantification. Here, we consider the problem of approximating the solution operator of the PDE, viewed as a map between subsets of infinite dimensional spaces. In recent years, several techniques based on neural networks (NN) have been developed to tackle this problem. In this talk, I will present some theoretical results on the approximation of solution operators of elliptic PDEs by NN-based surrogates. I will discuss the convergence rates of neural operators and how they vary depending on the smoothness of the coefficients in the input sets. I will then focus on PDEs with lognormal coefficients: in this case, the operator is not uniformly Lipschitz and the error is measured in the mean-square sense, with a Gaussian measure on the input space. The analysis of this case relies on (novel) sparsity estimates for the solutions and on the NN emulation of Hermite polynomials.