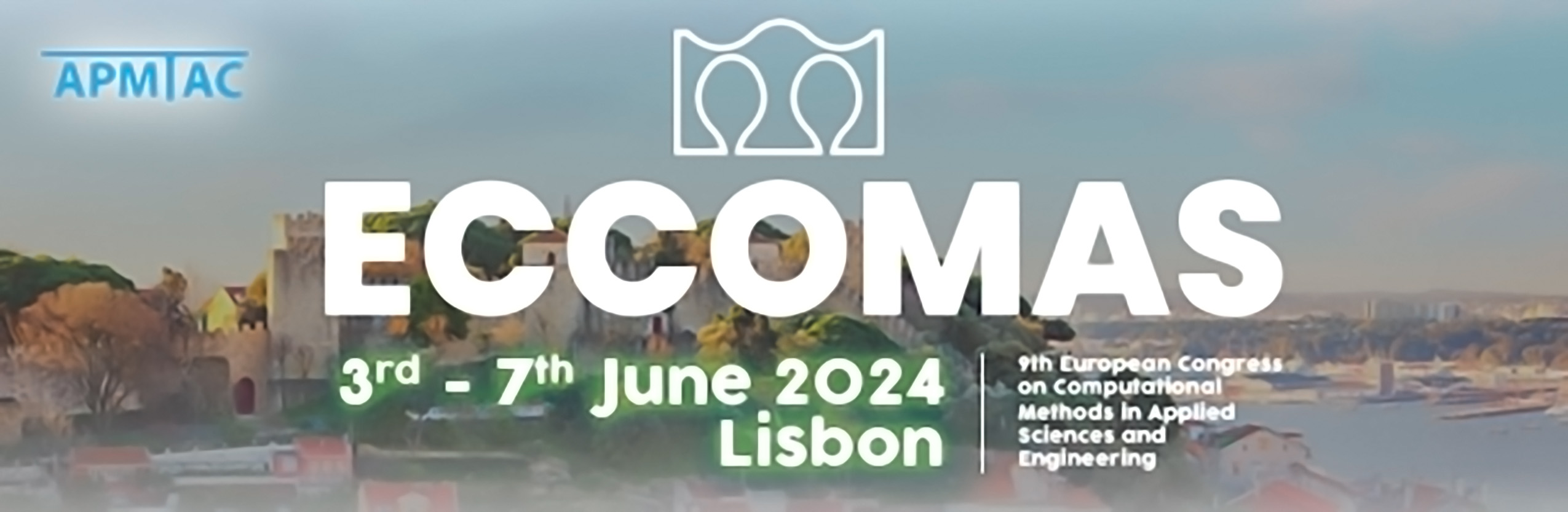
Turbulent separation bubble control using deep reinforcement learning in pre-exascale machines
Please login to view abstract download link
Deep reinforcement learning (DRL) for active flow control (AFC) has recently emerged as a promising alternative to classical control based on fluid-dynamics theory (Vignon et al. 2023). The ever-increasing computing power is closing the gap of incorporating scale-resolving fluid dynamics simulations into the DRL trial-and-error training loop. In this direction, we investigate the control efficacy of DRL in reducing a turbulent separation bubble (TSB) generated by and adverse-pressure gradient turbulent boundary layer. TSB is a naturally arising phenomenon in aircraft wings operating at high angles of attack, and an adequate control could yield safer maneuvers and a reduced fuel consumption. We find that the DRL agent is able to learn a successful control strategy eventually yielding a larger TSB reduction than classical periodic forcing control. Moreover, we assess the potential of transfer learning by comparing results from a DRL agent trained in both a coarse large-eddy simulation (LES) and a fine well-resolved LES. Finally, we introduce our open-source framework composed by the multi-GPU CFD solver SOD2D (Gasparino et al. 2023) and the DRL model implemented in SmartSOD2D, which also handles the communication between CFD solver and DRL agent using SmartSim (Partee et al. 2022). The natural nested parallelism of the training strategy, which consist in using multiple parallel CFD simulations feeding data into a single DRL agent, allows to properly harvest the computing power of pre-exascale machines.