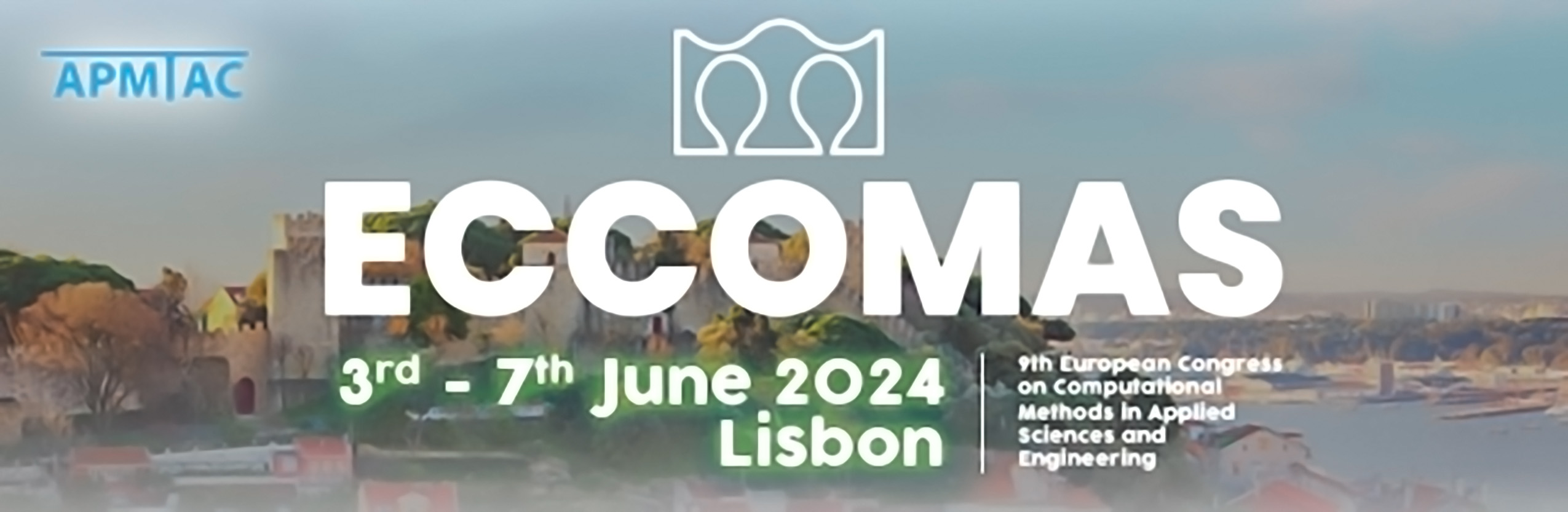
Machine Learning for Scientific Discovery: with Examples in Fluid Mechanics
Please login to view abstract download link
This work describes how machine learning may be used to develop accurate and efficient nonlinear dynamical systems models for complex natural and engineered systems. We explore several approaches to learn interpretable and generalizable dynamical systems that capture the essential “physics” of the system. We also discuss the importance of learning effective coordinate systems in which the dynamics may be learned. A theme throughout this work is that machine learning may be used to uncover new physics, and it is also possible to enforce known physics to improve machine learning algorithms. In engineering applications, these models may be integrated into a digital twin framework, where the models will be updated with new data. This opens up entirely new possibilities for active learning and control, which will also be discussed. We will demonstrate these learning approaches on a range of challenging modeling problems in fluid dynamics, and we will discuss how to incorporate these models into existing model-based control efforts. Because fluid dynamics is central to transportation, health, and defense systems, we will emphasize the importance of machine learning solutions that are interpretable, explainable, generalizable, and that respect known physics.