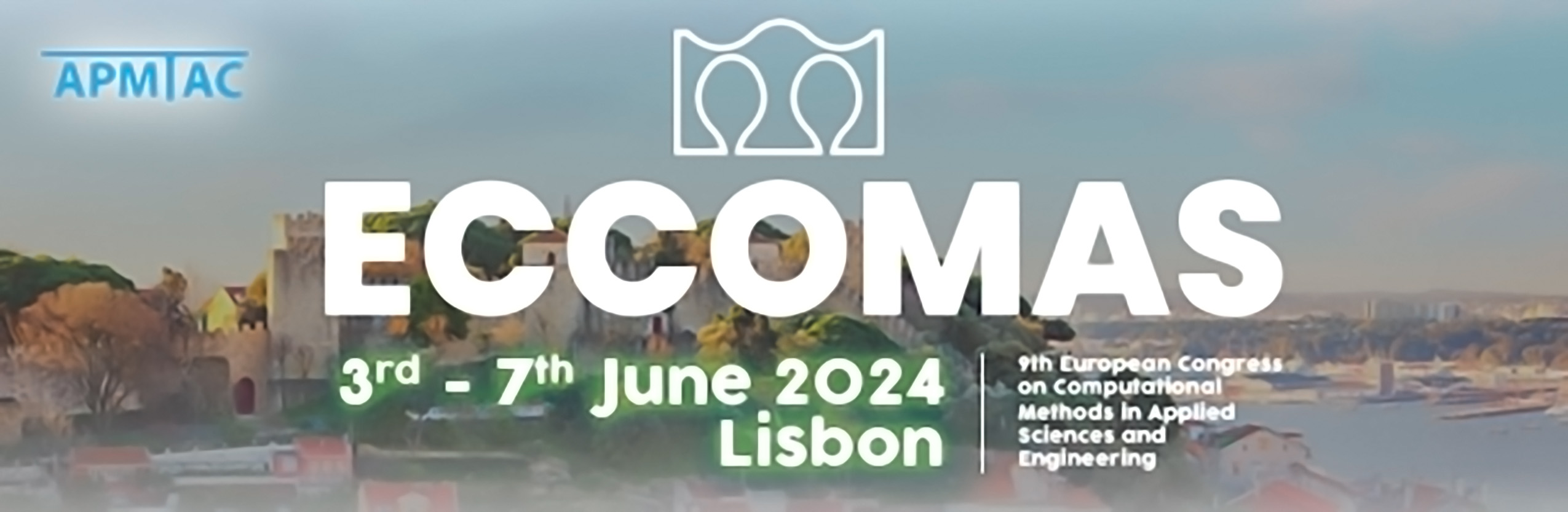
Automating Inverse Problems: A Differentiable Inverse Finite Element Solver for Elastography
Please login to view abstract download link
In vivo mechanical characterization of soft tissues using elastography finds applications in non-invasively detecting abnormal tissues and developing patient-specific simulation platforms. Elastography employs imaging modalities to measure tissue deformation induced by external stimuli. The extracted deformation field is then leveraged to estimate the material parameter field by solving an inverse problem posed as a PDE-constrained optimization problem. The gradient-based optimization techniques are used for solving such problems wherein the difference between experimentally observed and model-computed deformation fields (parameterized by the latest estimate of material parameter field) is minimized iteratively. However, accurately and efficiently computing the gradient of the objective function with respect to the model parameters is challenging. Although the adjoint method mitigates computational costs, analytically deriving various implicit terms arising in gradient computation is non-trivial due to the complex nonlinear constitutive models used. Differentiable simulation has emerged as a promising avenue to automate the entire process of solving such inverse problems. This approach allows for representing the numerical solution procedure as a computational graph, which can be differentiated using Automatic Differentiation (AD). This enables efficient computation of gradients, an essential requirement for any gradient-based optimization solvers. The present work demonstrates the application of a differentiable inverse Finite Element (FE) framework for solving inverse problems of quasi-static and dynamic elastography. The framework employs a differentiable FE solver to solve the PDEs and efficiently compute the gradients using AD-based adjoint methods. The framework reports a 30% decrease in computational effort compared to a MATLAB-based solver. The differentiable FE-based framework opens avenues for enhanced sensitivity analyses and the application of machine learning techniques in scientific simulations, engineering design, and computational research.