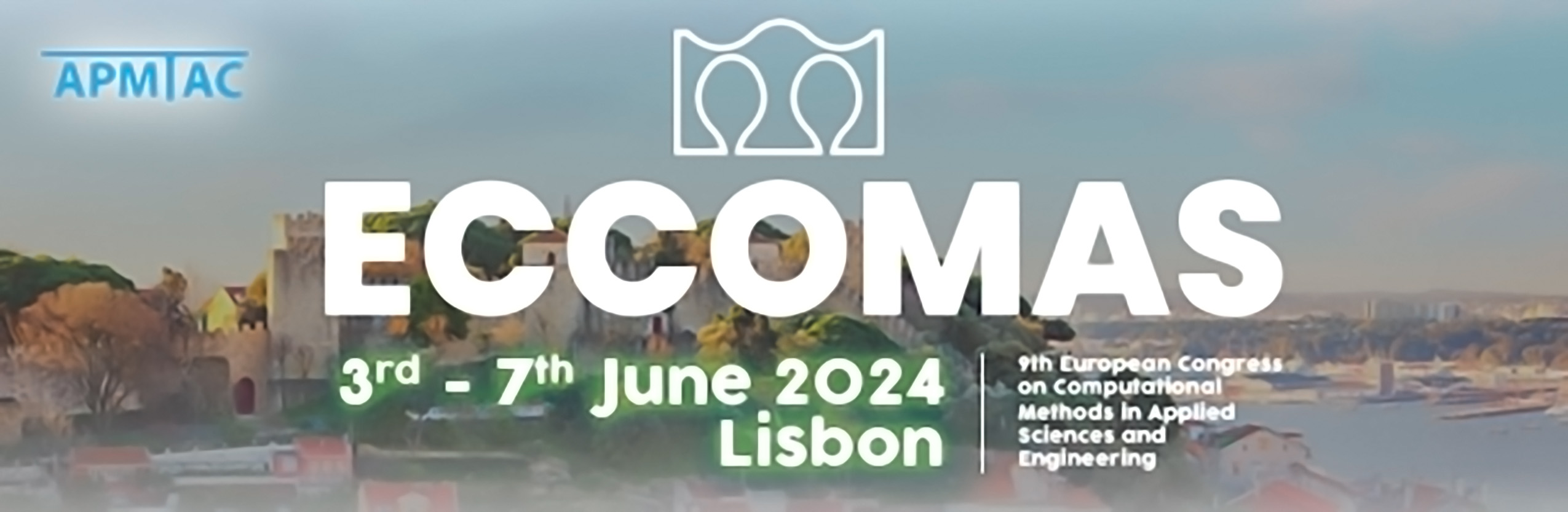
Harnessing Generative AI for Modelling Mesoporous Materials: An Aerogel Use Case
Please login to view abstract download link
Mesoporous materials exhibit immense potential across various domains, from energy storage to thermal superinsulation, thereby making them attractive for materials development with a focus on the planet's green energy objectives. Among these mesoporous materials, silica aerogels have been gaining attention, due to their very low thermal conductivity and low density making them suitable as (high-temperature) thermal superinsulation. Traditional lab-based iterative materials development is time and resource intensive, often generating substantial waste. However, the advent of machine learning and computational modelling has facilitated the expedited development of such complex materials. In this study, a computational methodology for precise image-based reconstruction of mesoporous microstructures is presented, harnessing the capabilities of Convolutional Neural Networks (CNNs) synergistically with generative deep learning algorithms, specifically the conditional Generative Adversarial Network (cGAN). While conventional GANs have found application in materials reconstruction within the scientific literature [1], this research uniquely capitalises on CNNs to discern microstructural attributes from a 2D image slice, thereby utilising them as conditioning parameters for the cGAN. This approach serves to mitigate the inherent stochasticity of GAN outputs, leading to deterministic microstructures. Consequently, the resultant 3D microstructure can be effectively employed for comprehensive multi-scale computational analysis of structure-property relationships. This advancement facilitates expeditious material development, eliminating the necessity for repetitive experimental synthesis for characterisation purposes. REFERENCES [1] A. Henkes and H. Wessels. Three-dimensional microstructure generation using generative adversarial neural networks in the context of continuum micromechanics, Computer Methods in Applied Mechanics and Engineering, 400: 115497, 2022