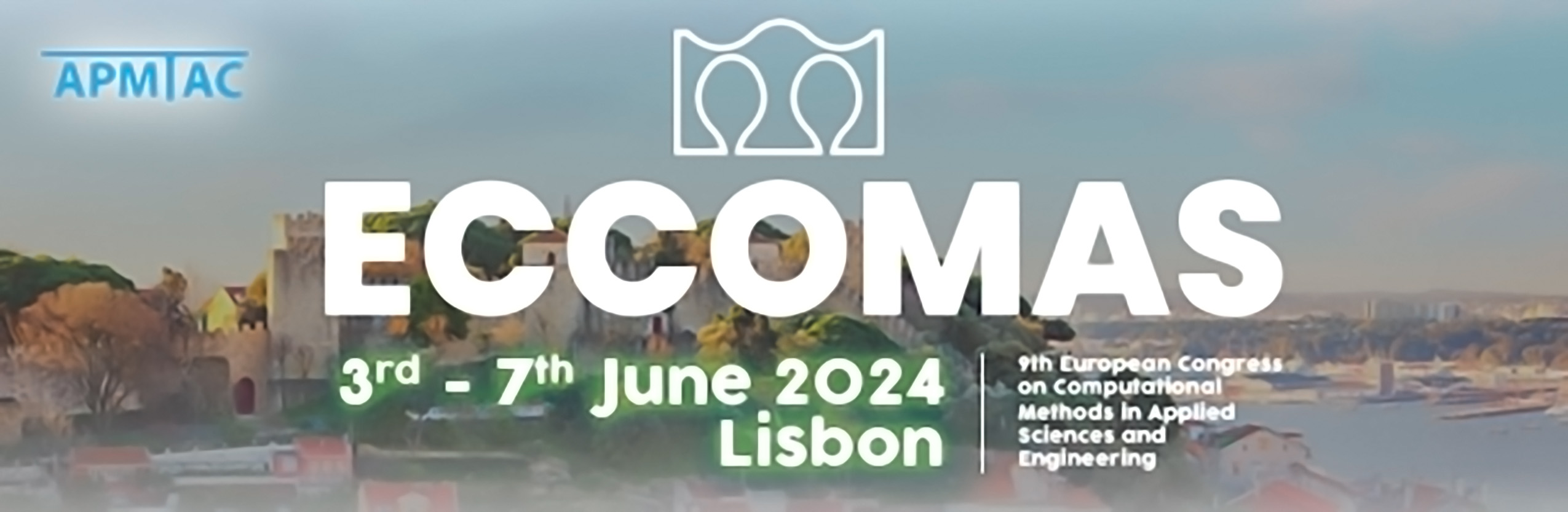
Large-scale Statistical Learning for Mass Transport Prediction in Porous Materials Using 90,000 Artificially Generated Microstructures
Please login to view abstract download link
Effective properties of functional materials crucially depend on their 3D mi- crostructure. In the present contribution, quantitative relationships between descriptors of two-phase microstructures and their mass transport properties are investigated. For this purpose, a vast database comprising 90,000 microstructures drawn from nine different stochastic models is generated by using various methods from stochastic geometry. For each structure, the resulting effective diffusivity and permeability as well as various microstructural descriptors are computed. To the best of our knowledge, this is the largest and most diverse dataset created for studying the influence of 3D microstructure on mass transport. In particular, microstructure-property relationships are established using analytical prediction formulas, artificial (fully-connected) neural networks and convolutional neural networks. For details, see [1]. The corresponding data is available online [2]. [1] B. Prifling, M. Röding, P. Townsend, M. Neumann, and V. Schmidt, “Large-scale statistical learning for mass transport prediction in porous materials using 90,000 artificially generated microstructures” Frontiers in Materials, vol. 8, 786502, 2021. [2] B. Prifling, M. Röding, P. Townsend, M. Neumann, and V. Schmidt. doi:10.5281/zenodo.4047774, 2021