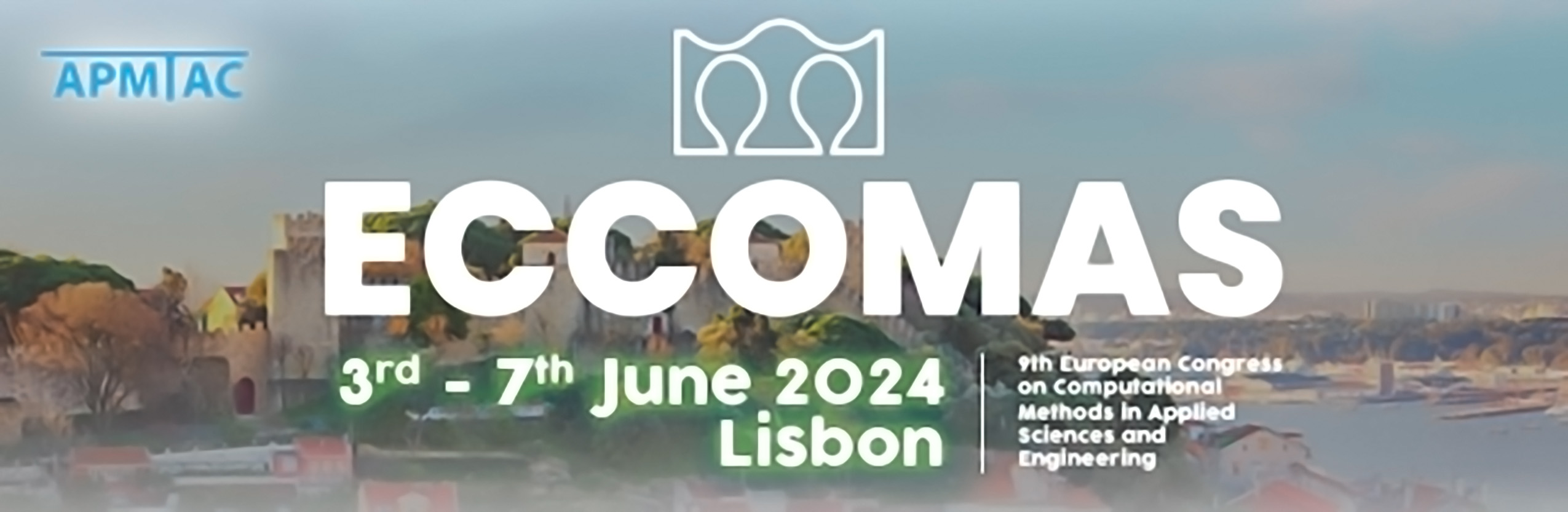
Structure-Preserving Adaptive Hyper-Reduction of Parametric Hamiltonian Systems
Please login to view abstract download link
It is well known that model order reduction of non-linear dynamical systems does not typically result in significant speedups, since the computational cost of evaluating non-linearities still depends on the dimension of the full order problem. In the case of (parameterized) Hamiltonian systems, standard hyper-reduction techniques such as the empirical interpolation method (EIM) fail to preserve the geometric structure of the original problem, as the approximation of the non-linear Hamiltonian gradient is not a gradient in general. As an additional challenge, non-dissipative phenomena like transport-dominated problems and wave propagation do not possess a global low-rank structure, meaning that large approximation spaces are necessary to achieve even moderate accuracy. In order to address these issues, we present an adaptive, structure-preserving hyper-reduction scheme where the EIM basis and interpolation points are updated over time. A parameter sampling strategy is developed, so that the computational complexity of adapting the EIM space does not depend on the product of the full order dimension and the number of test parameters. We will also discuss how to update the dimension of both the reduced space and the hyper-reduction space to account for variations in the numerical rank of the state.