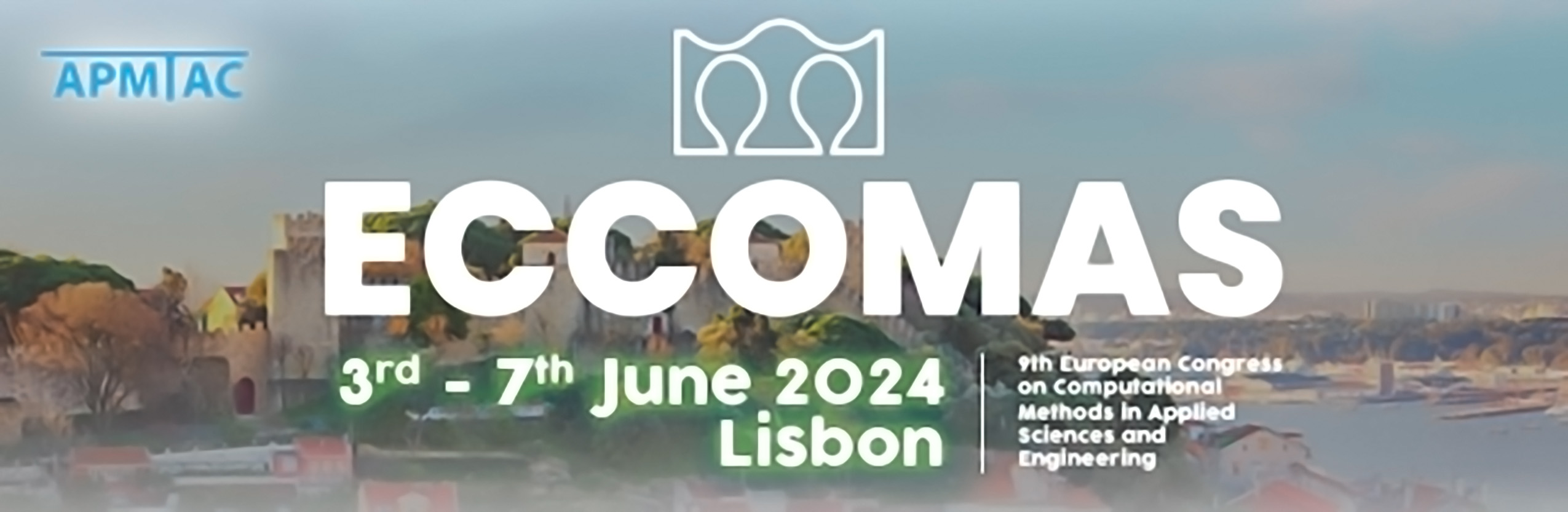
r-Adaptive Deep Learning PDE Solver With Triangular Meshes
Please login to view abstract download link
The $r-$adaptive deep learning method [1] is a novel mesh-based method to solve Partial Differential Equations (PDEs). It uses Deep Neural Networks (DNN) to optimize the mesh and the solution of the PDE simultaneously. However, in our previous work [1], we restricted to tensor product meshes due to the lack of a triangular mesh generator with access to its derivatives with respect to the node locations. Herein, we implemented an open-source triangular mesh generator with derivatives computed via automatic differentiation. It allows to mesh non-convex domains and mark border nodes to consider different boundary conditions. By including the mesh generator as a layer of the DNN, the topology of the mesh can be modified during training, in contrast to traditional mesh-moving algorithms where the topology remains invariant. To numerically illustrate the performance of the $r$-adaptive method using triangular meshes, we analyze the convergence of the method --in combination with the Deep Ritz Method [2]-- over smooth PDE solutions, singular ones, and some exhibiting strong gradients. We also show the evolution of the meshes during the training process. [1] Á.J. Omella and D. Pardo. r-Adaptive deep learning method for solving partial differential equations. Computers & Mathematics with Applications, Vol. 153, pp. 33-42, 2024. [2] W. E and B. Yu. The Deep Ritz method: A Deep Learning-Based Numerical Algorithm for Solving Variational Problems. Comput. Methods Appl. Mech. Eng., Vol. 6, pp.1-12, 2018.