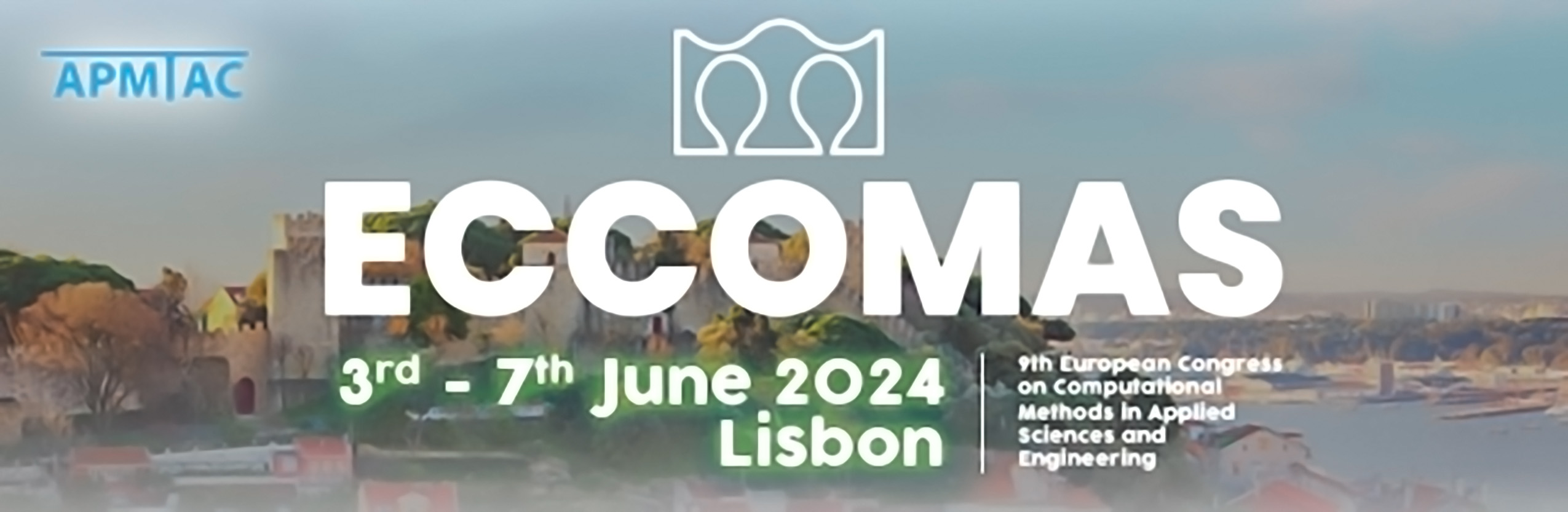
Structural optimization for fail-safe designs by machine learning
Please login to view abstract download link
Due to its efficiency-orientated nature, designs obtained by “conventional” topology optimization are susceptible to localized stiffness loss caused by material failure. In certain safety-critical applications, it is imperative to design structures that remain functional even if a structural member is damaged. To address this challenge, Jansen et al. [1] proposed an approach for fail-safe topology optimization. The resulting design includes redundant load paths that significantly increase its robustness to local material losses. Parallelization of the finite element method can reduce computation time, but the problem remains extremely computationally intensive. More efficient approaches either do not consider fail safety explicitly, or restrict the design freedom [2]. A new method utilizing an artificial neural network (ANN) is presented to address the computational challenges associated with optimization for fail safety. This method aims at generating fail-safe designs with redundant load paths from designs created using “conventional” topology optimization. To achieve this, an autoencoder is trained to map the density field of a “conventional” design to a corresponding fail-safe design. The dataset for the supervised training process is created considering numerous combinations of design spaces, boundary conditions, loads, and volume fractions. For each combination, a "conventional" and a fail-safe topology optimization is performed, focusing on compliance. The backpropagation algorithm utilized for the training, minimizing the pixel error between the "conventional" and fail-safe design. The resulting ANN is capable of accurately predicting simple fail-safe designs that include redundant load paths and achieve comparable compliance values. Nevertheless, as the complexity increases, e.g. due to the consideration of additional design spaces, boundary conditions or loads, the quality degrades rapidly. This decline manifests in disconnected load paths and noisy designs, rendering them inapplicable. The current contribution presents the potentials and limitations of the described approach and discusses possible strategies to overcome current limitation in order to enable the fail-safe approach to be applied to non-academic applications.