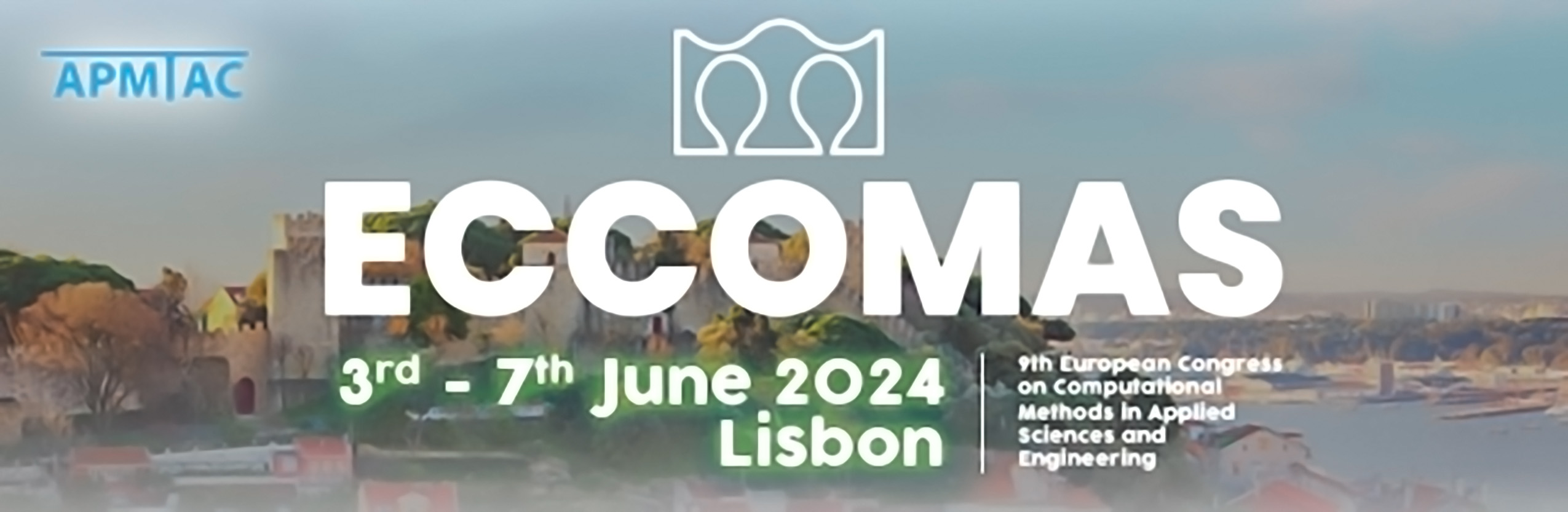
Proper Generalized Decomposition for Topology Optimization of Problems with Separable Geometry
Please login to view abstract download link
Many applications of density-based topology optimization require a very fine mesh resolution, either to obtain high-resolution designs, or to resolve physics in sufficient detail. Solving the discretized state and adjoint Partial Differential Equations (PDEs) in every iteration step becomes computationally demanding, restricting the applicability of the method. Model Order Reduction (MOR) offers a solution for this computational burden, using a reduced vector basis for simulations and resulting in a higher computational speed and reduced storage requirements. In scenarios where the multi-coordinate density field can be expressed as a sum of products of lower-coordinate basis functions, one potential MOR technique is Proper Generalized Decomposition (PGD). PGD computes the basis functions on-the-fly as the problem is solved, making it an a priori method. It does not require prior knowledge of the solutions, omitting the need for full system solves, unlike a posteriori methods as Proper Orthogonal Decomposition. Over the last few years, PGD has been successfully used for the solution of various types of PDEs [1], but it has not yet been applied for topology optimization. To explore the potential of the method in the context of topology optimization, we use PGD for the optimization of a 3D ribbed floor for minimum compliance. The geometry of a ribbed floor can be expressed as a sum of products of 2D functions for the in-plane coordinates (representing the rib pattern), and 1D functions for the thickness coordinate (representing the distinction between compression slab and ribs). The separated PGD approximation is obtained with a method similar to the subspace iterations used for eigenproblems. Numerical tests show significant increase of computational efficiency. To further refine the method, we explore various improvements. The first involves recycling information from prior iterations as an initial guess for the PGD approximation algorithm. Additionally, we investigate a ‘one-shot’ approach, where the approximation is obtained with only one PGD iteration for every optimization step. Numerical tests yield a decrease in calculation time ranging from 100 to 250 times compared to the full approach. Our findings showcase the potential of PGD as a powerful tool in topology optimization of problems with separable geometry, offering substantial gains in computational efficiency without compromising solution accuracy.