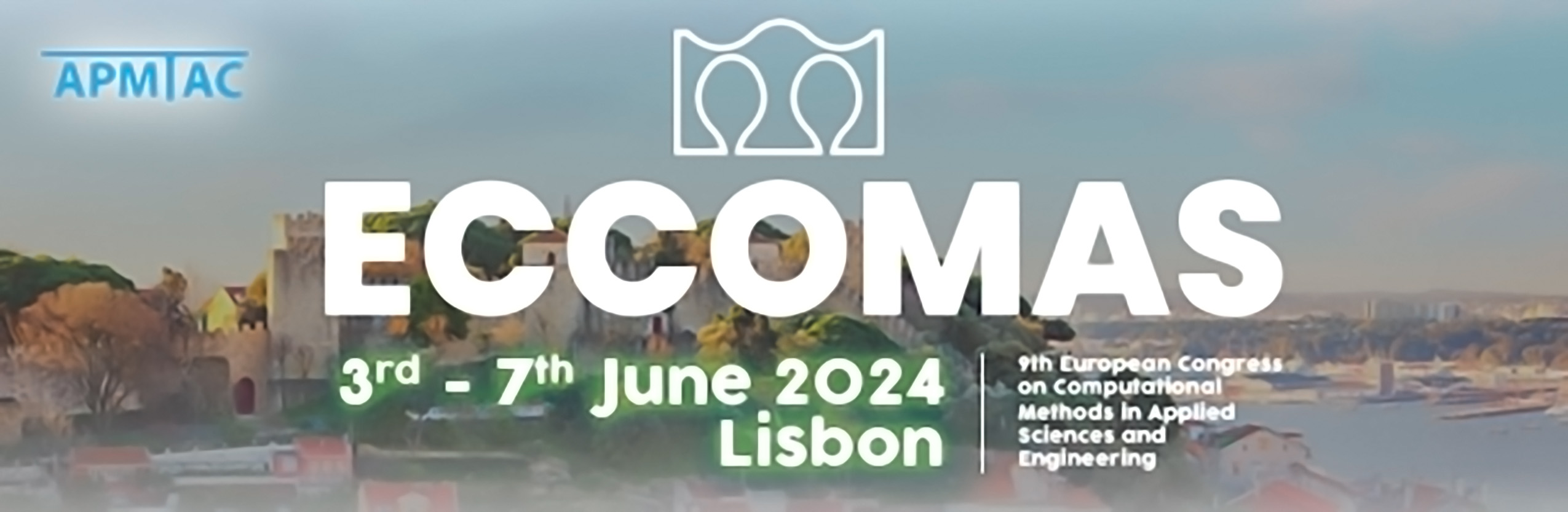
High-Fidelity Computational Fluid Dynamics and Particle Modelling Using Neural Networks
Please login to view abstract download link
Recent developments in AI are transforming a large number of fields and are now starting to make a major impact in computational physics. Here we describe some of these innovative AI techniques that have been recently developed and how they can work together. We will indicate how AI may be deployed for modelling of environmental flows including indoor and outdoor flows and air water systems e.g. flooding. Recent advances have enabled AI software to solve, to within numerical tolerances, the discrete differential equations that govern the physics of fluids (AI4PDEs) [1, 2]. Important also is the use of AI to solve particle systems (e.g. AI for Discrete Element Modelling (AI4DEM)) which are expressed by interparticle forces and Lagrangian particle motion. The presentation will provide a summary of these forward models and a view on how these new approaches can be used with trained AI models to form even more powerful methods. REFERENCES [1] B. Chen, C. E. Heaney and C. C. Pain. Using AI libraries for Incompressible Com- putational Fluid Dynamics, in preparation (2024). [2] B. Chen, C. E. Heaney, J. L. M. A. Gomes, O. K. Matar and C. C. Pain. Solving the Discretised Multiphase Flow Equations with Interface Capturing on Structured Grids Using Machine Learning Libraries, arXiv preprint (2024). arxiv.org/abs/2401.06755