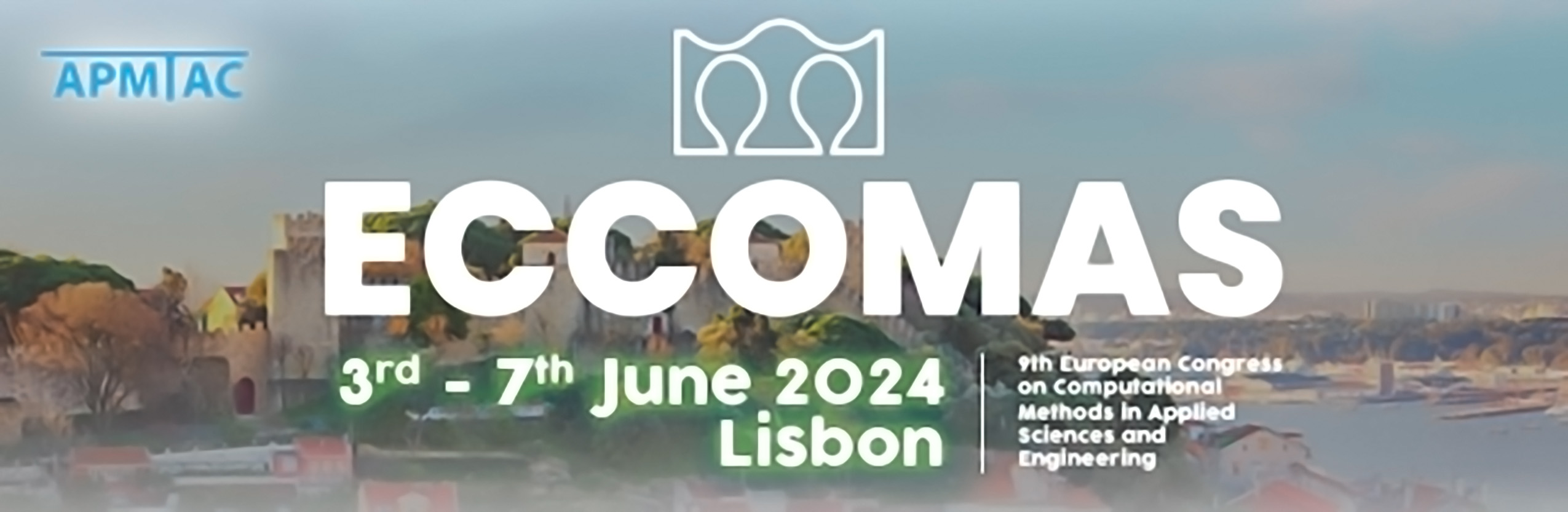
Review and challenges for a realistic numerical SHM benchmark
Please login to view abstract download link
This paper presents a comprehensive review and critical discussion of existing numerical benchmarks in Structural Health Monitoring (SHM), focusing on the challenges and limitations of accurately simulating real-world conditions. The review presents the evolution of benchmarks from simplistic models to more complex simulations that aim to replicate operational and environmental variabilities (EOVs) and various damage scenarios. Despite these advancements, benchmarks currently available in the literature have yet to incorporate additional phenomena, such as long-term environmental effects and sensor faults or malfunctions. A proposal for a new benchmark integrating these factors into the simulation process is presented in the paper. This benchmark aims to facilitate comprehensive testing and validation of SHM techniques in a controlled, realistic numerical setup by including both fast and slow-varying damage scenarios and sensor malfunctions. The proposed framework is applied to a simple structural model—a steel beam subject to varying loads and environmental conditions—demonstrating its potential to simulate a wide range of real-world phenomena.