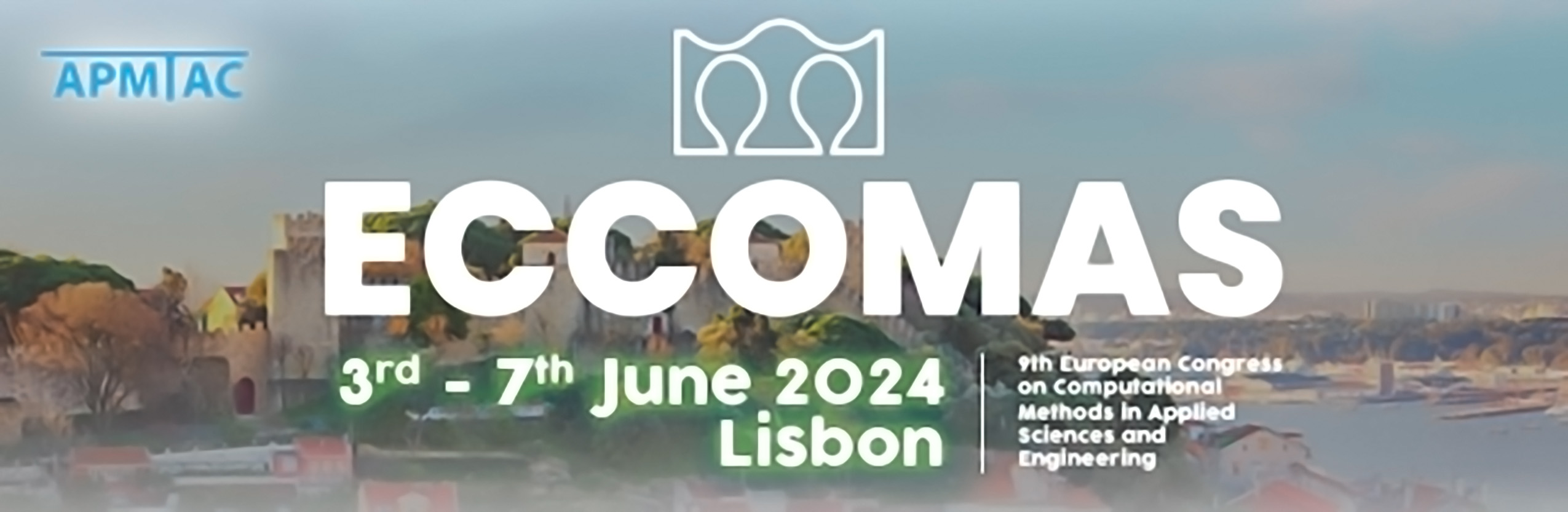
Aerodynamic predictions under uncertainty using Autoencoders
Please login to view abstract download link
With the increasing popularity of Machine Learning (ML) and, particularly, autoencoder (AE) techniques, it becomes crucial to evaluate the confidence level that can be attributed to the generated results. Psaros et al. (2023) provide insightful reviews on Uncertainty Quantification (UQ) methods for deep learning techniques, highlighting persistent challenges in the field. UQ for AEs remains an area with limited coverage in the literature, with Variational AEs being a prevalent approach. The present work operates within the ensemble framework, which have demonstrated exceptional performance in a few studies, yet the relationship between performance, confidence measures, and different uncertainty sources remains unclear. We employ ensembles of convolutional AEs for predicting flow fields around wing sections; demonstrating the ability of AE ensemble to effectively discriminates between diverse sources of uncertainty: internal (model-form) and external uncertainties. The former is due to limited or noisy data, hyperparameters choices and network architecture, while the latter is a consequence of limited knowledge of the operating conditions in the prediction scenario. Our approach yields predictions with confidence intervals accurately reflecting the relative importance of various sources of uncertainty. Additionally, the standard deviation of the ensemble’s predictions proves to be a reasonable proxy for actual error, a crucial feature for the safe integration of ML algorithms into real-world applications. Results obtained from the ensemble of AEs are compared with those derived from Gaussian Process Regression, showcasing distinctions and highlighting the superior capability of AEs in discerning high- and low-confidence prediction scenarios in the absence of ground truth data.