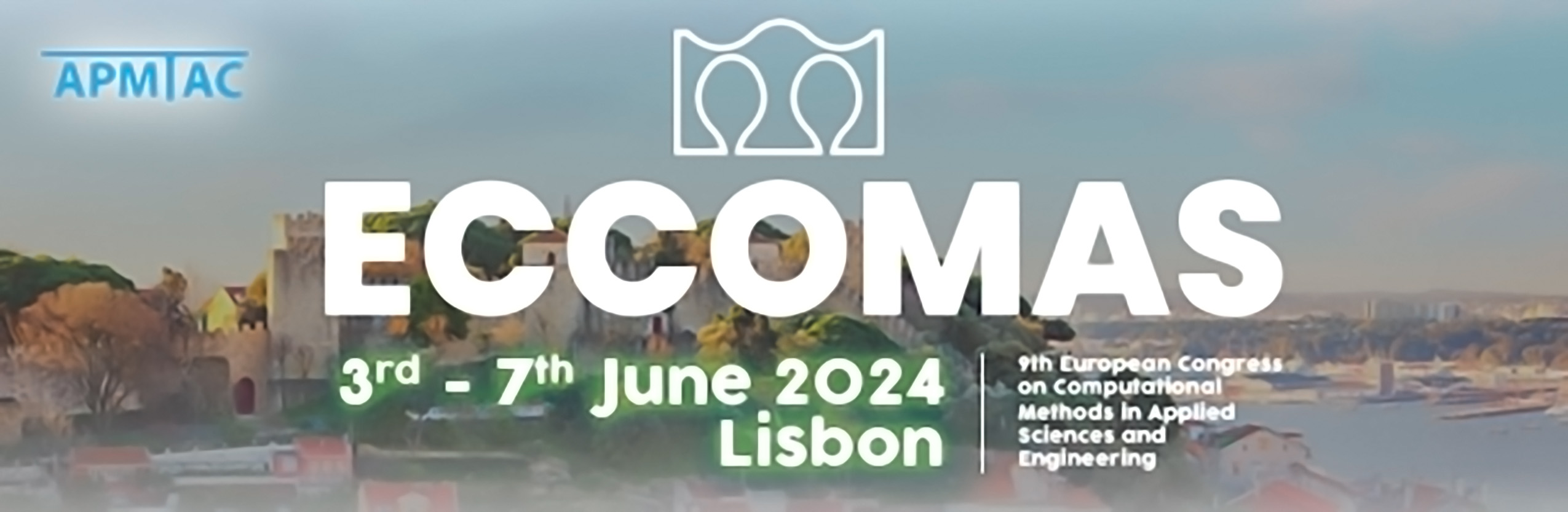
Physics-Informed Neural Networks as surrogate for Robust Design Optimization
Please login to view abstract download link
This contribution introduces a novel framework that integrates Physics-Informed Neural Networks (PINNs) into the domain of Robust Design Optimization (RDO). The surrogate model based on PINNs leverages the computational efficiency inherent in PINNs for the repetitive analysis of fundamental solution within RDO. The PINNs are trained to optimize the loss term derived from the data generated in Finite Element Method (FEM) simulations as well as the loss terms obtained from boundary conditions, the strong form, and the weak form of the constitutive description. Notably, these PINNs incorporate the design parameters of RDO as input variables, subsequently subject to optimization. The resultant output serves as the basis for formulating the objective function for optimization. The design parameters in the optimization task and a priori are presented considering polymorphic uncertainty. Polymorphic uncertainty is treated through the utilization of fuzzy probability-based randomness. A comprehensive sensitivity analysis is undertaken to identify the critical design parameters. The performance evaluation of PINNs is systematically conducted across various permutations of discrete and continuous design parameters in the input space. Finally, the application and capabilities of the developed framework is illustrated through an example.