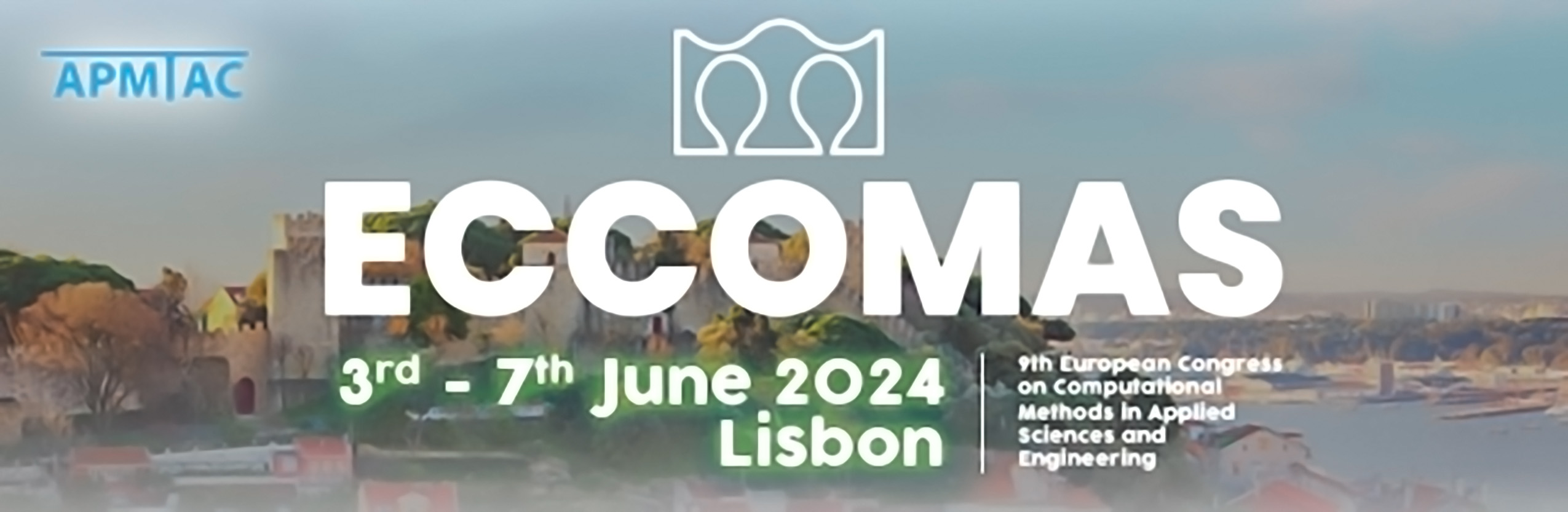
A physically recurrent neural network for rate dependent composite materials
Please login to view abstract download link
Machine learning techniques have shown great potential for reducing the computational cost of numerical analysis based on finite elements (FE). For instance in computational homogenization, so-called surrogate models can replace the FE problem at the microscale - the main computational bottleneck of the method. However, applying these models to predict the microscopic behavior of complex materials with history-dependence comes with challenges. Two well-known problems in one of the most popular methods, Recurrent Neural Networks, are their poor extrapolation properties and their data-hungry nature. The alternative proposed in this work, the Physically Recurrent Neural Network (PRNN) [1], addresses those problems by incorporating knowledge of classical constitutive models into a data-driven surrogate. Here we extend the PRNN to deal with rate and path-dependent heterogeneous materials in a 3D finite strain framework. Polar decomposition is applied to obtain stretch and rotation from the deformation gradient. Then, the network is used to learn the mapping between stretch and unrotated stress, from which the stress in the global coordinate is retrieved using the principle of material objectivity. In the network, we encode the homogenized stretch into a set of deformation gradients passed to a set of fictitious material points where the stress is computed using the same material models and properties as in the micromodel. With a history-dependent model, another valuable output is obtained: the internal variables. These are updated at every load step by the material model and work as the physics-based memory of the network. To obtain the homogenized stress, a decoder is applied to the material points stresses. The PRNN is employed as the surrogate model for a unidirectional composite micromodel with rate-dependent plasticity in the matrix and hyperelasticity in the fibers. The network's performance is first assessed for a single micromodel, in which we showcase its ability to extrapolate to different strain-rates and unloading/reloading scenarios. Then, we apply it to a multiscale problem with off-axis loading.