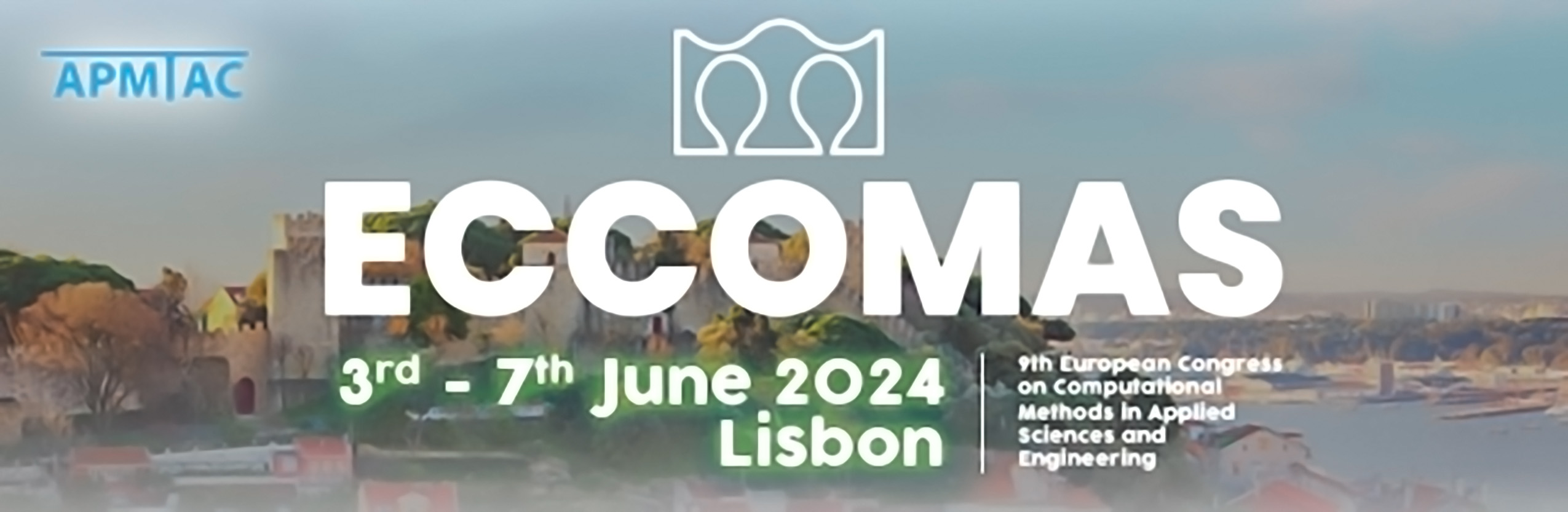
Tensor Train Representation for Efficient Modeling of High-Dimensional Flamelet-Based Manifolds in High-Speed Propulsion Simulations
Please login to view abstract download link
Finite-rate chemistry (FRC) based computational fluid dynamic (CFD) simulations em- ploying detailed chemical mechanisms provide high-fidelity chemistry representation but demands a computationally intensive effort, particularly for mechanisms with thousands of reactions and hundreds of species. The challenge is exacerbated by the stiffness of chem- ical source terms due to disparate time scales of chemical reactions, leading to potential numerical instability. In contrast, flamelet-based combustion models offer cost-effective solutions for scale-resolved simulations with detailed chemical mechanisms. However, they necessitate higher-dimensional flamelet manifolds to accurately predict the under- lying physics in high-speed propulsion scenarios. Yet, computational complexity grow exponentially with increasing table dimensionality, known as the curse of dimensionality. This study builds upon our previous work [1], in which, for the first time, the applica- bility of tensor trains (TTs) as an efficient alternative to the artificial neural network (ANN)-based flamelet approaches for representing high-dimensional flamelet manifolds was demonstrated. The TT representation decomposes the multi-dimensional flamelet data into low-rank tensors, facilitating the efficient approximation, and reduces the computational complexity from exponential to linear scaling. The implementation of TT framework, in a solver provided by the JENRE Multiphysics Framework yielded two or- ders of magnitude reduction in memory requirements for a 5D flamelet table. The TT framework not only closely reproduced the results of the base flamelet table within accept- able error margins in 2D CFD simulations but also showcased the TTs as a highly effective means of representing source term. This stands in sharp contrast to ANNs, which often face challenges in accurately predicting such intricate details. In this work, we extend the demonstration of TT’s applicability and efficiency to flamelet tables exceeding 10 GB of memory and analyze the accuracy of TTs by means of 3D reacting CFD simulations.