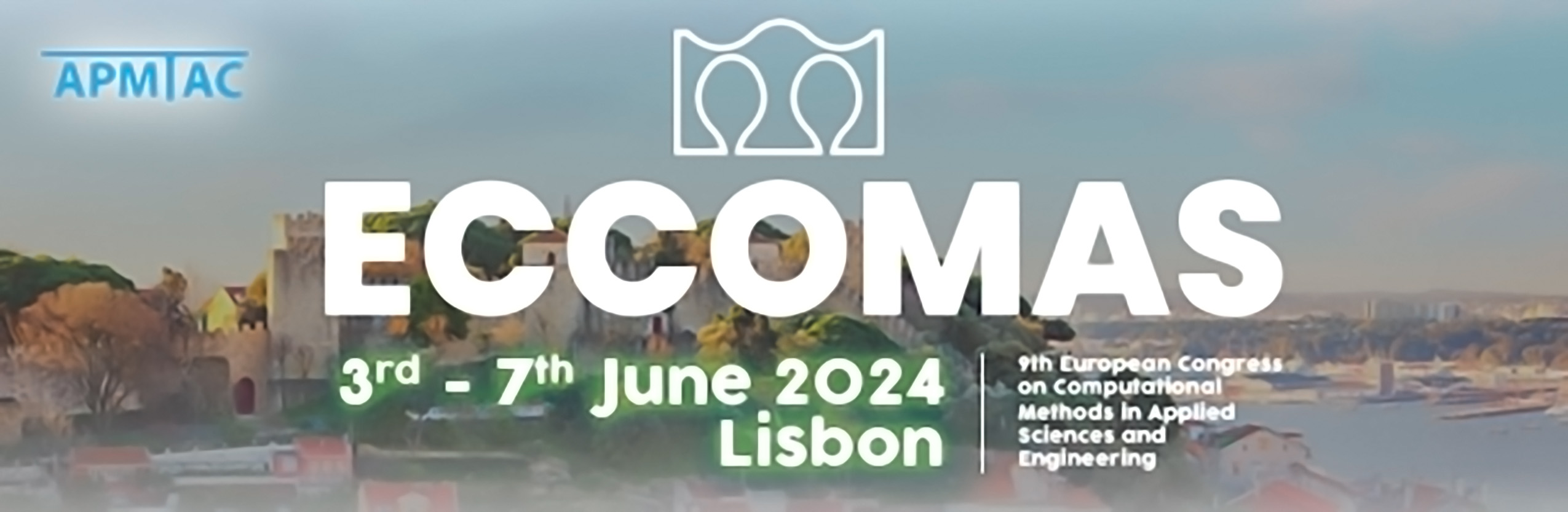
A Deep Learning Surrogate Model for Reservoir Characterization
Please login to view abstract download link
The safety and environmental impacts of subsurface resource exploitation demand ro- bust geomechanical modeling. However, uncertainties arising from model assumptions, intrinsic parameter variability, and data errors challenge prediction reliability. Sensitivity analysis and uncertainty quantification are crucial to address these issues. In com- plex, large-scale settings, inverse modeling and stochastic data assimilation methods offer reliable solutions but often at a significant computational cost. To tackle these challenges, this work develops a Neural Network (NN)-based proxy model, that leverages laboratory tests and in-situ observations to approximate geomechanical results. Specifically, the NN-model is trained on snapshots generated by a full-order model, making it available for real-time or multi-query applications where rapid computation is of paramount importance. Moreover, by constraining predictions to adhere to physical laws, the method can ensure not only computational efficiency but also physical consistency. The boost of physical knowledge will also reduce the need of data for a successful training, thus possibly limiting the curse of dimensionality issue. The objective of this work is to integrate deep learning with traditional modeling activities in the geomechanical analysis of deep reservoir applications. This integration can enhance prediction reliability, offering robust simulations crucial for design and optimization of the reservoir management program. Such approach can represent a step toward more accurate and efficient subsurface resource management, fostering sustainable practices and minimizing environmental risks.