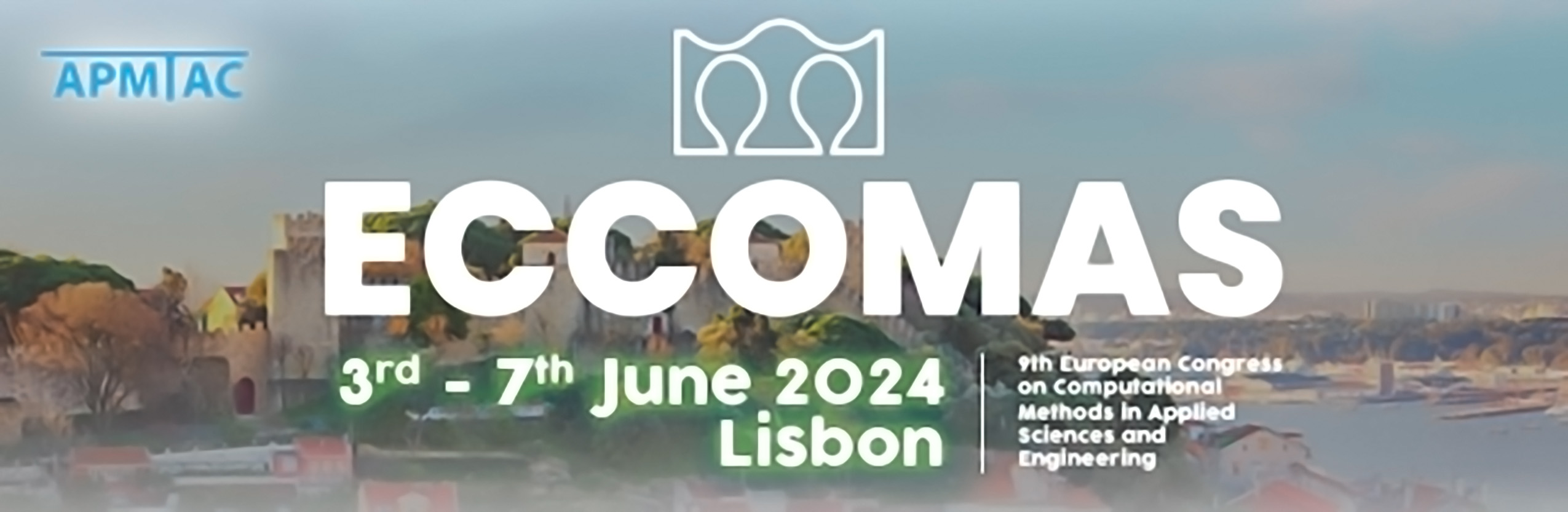
Current Progress on the Appromation of Hyperbolic Balance Laws Using Physics-Informed Neural Networks (PINNs) and Weak PINNs (WPINNs)
Please login to view abstract download link
The talk aims to present our current progress on the approximation of a specific class of partial differential equations (PDEs) known as hyperbolic balance laws which describes the conservation of mass, momentum, and energy in many physical systems like shallow-water system, compressible Euler equations, among others. In this talk we investigate the use of PINNS [1] and Weak PINNS [2] for the approximation of 1D hyperbolic balance laws. One of the main difficulties when dealing with hyperbolic systems is the lack of regularity of the solutions, that may present discontinuities that propagates at finite speed. Another important issue when dealing with balance laws is the presence of non-trivial steady states. A huge literature has been developed to design of numerical schemes, that are named as well-balanced methods, that are able to correctly simulate the propagation of small perturbation around those steady states (see [3] and the references therein). Moreover, in the hyperbolic framework is also important to construct numerical methods that are able to select the correct entropy solutions. Recently [2] a wPINNS formulation that introduces the entropy inequality has been proposed to stabilize the learning process and guide it towards approximating the correct weak solution more effectively. In this work we compare standard PINNS where some viscous regularization has been proposed to improve the learning process and that is able to accurately recover some relevant steady states of the balance laws. Comparisons with wPINNS that incorporates the entropy regularization is also provided. Finally some numerical results for the 1D shallow-water system with bathymetry and manning friction are also performed.