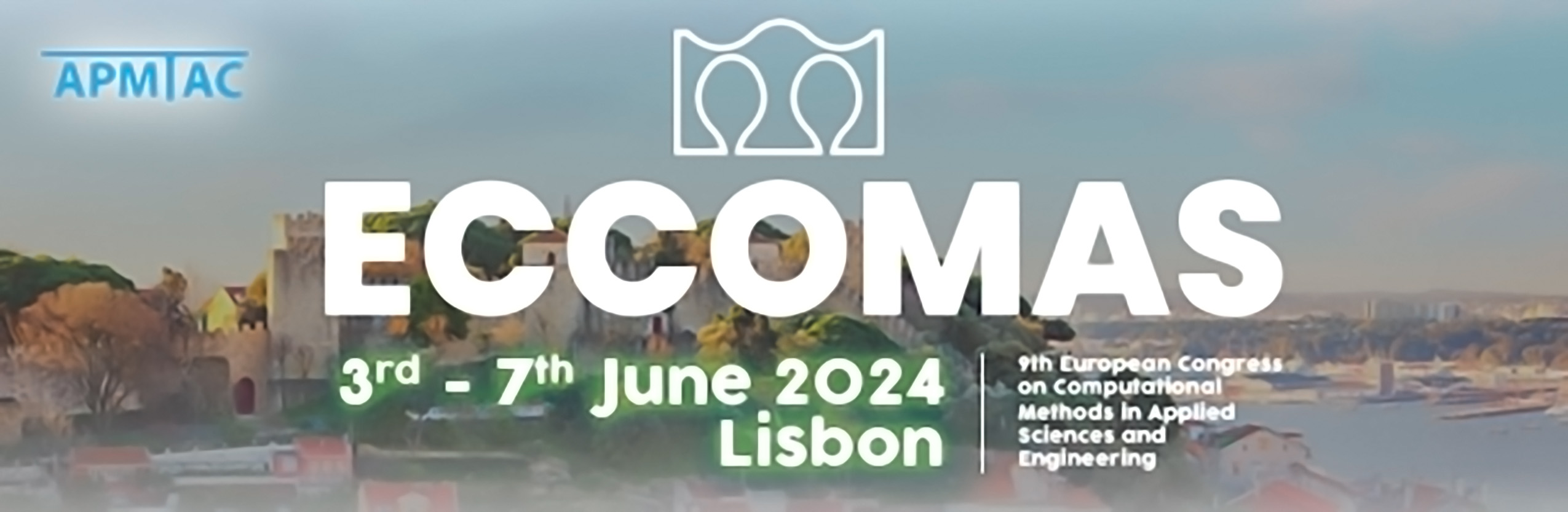
Phase-Field Modeling Of Fracture Processes Using Physics-Informed Deep Learning
Please login to view abstract download link
Phase-field fracture modelling [1] recasts the problem of fracture as a variational problem which completely determines the fracture processes including crack nucleation, propagation, bifurcation, and coalescence, and obviates the need for ad-hoc conditions. In this approach, a phase field is introduced in the formulation which regularizes a crack. It is however a nonlocal model which includes a small length scale. Resolving this length scale in computation is expensive. Hence, uncertainty quantification, material parameter identification, design optimization, among others, using this approach become prohibitively expensive. Recent advances in deep learning for applications in scientific modeling and computation [2] provide promising pathways to resolve this challenge. We explore their application to phase-field fracture modeling within the framework of physics-informed neural networks (PINNs). Since phase-field fracture is governed by a nonconvex variational energy, we employ Deep Ritz method (DRM) [3] in which the solution field is represented by a neural network (NN) and the training of the network proceeds by directly minimizing the variational energy of the system. We solve some benchmark problems in phase-field modeling of crack nucleation, propagation, branching, and coalescence using deep learning. We first study crack nucleation in a 1-D homogeneous bar with prescribed end displacements. Using this example, we elucidate the challenges in obtaining solution using NNs with the same level of domain discretization as needed in finite element analysis. Then we discuss crack nucleation in an L-shaped panel and point to the challenges that NNs face in learning the nucleation of a crack in 2-D. We also study crack propagation and branching in a single edge notched plate under tension and shear loading. The presence of spurious energy minima makes it challenging to obtain the correct solution when prescribed displacement is not purely tensile. Finally, we show that appropriately designed NNs can learn crack coalescence as well. The details of the models and the challenges in obtaining the correct solution will be discussed.