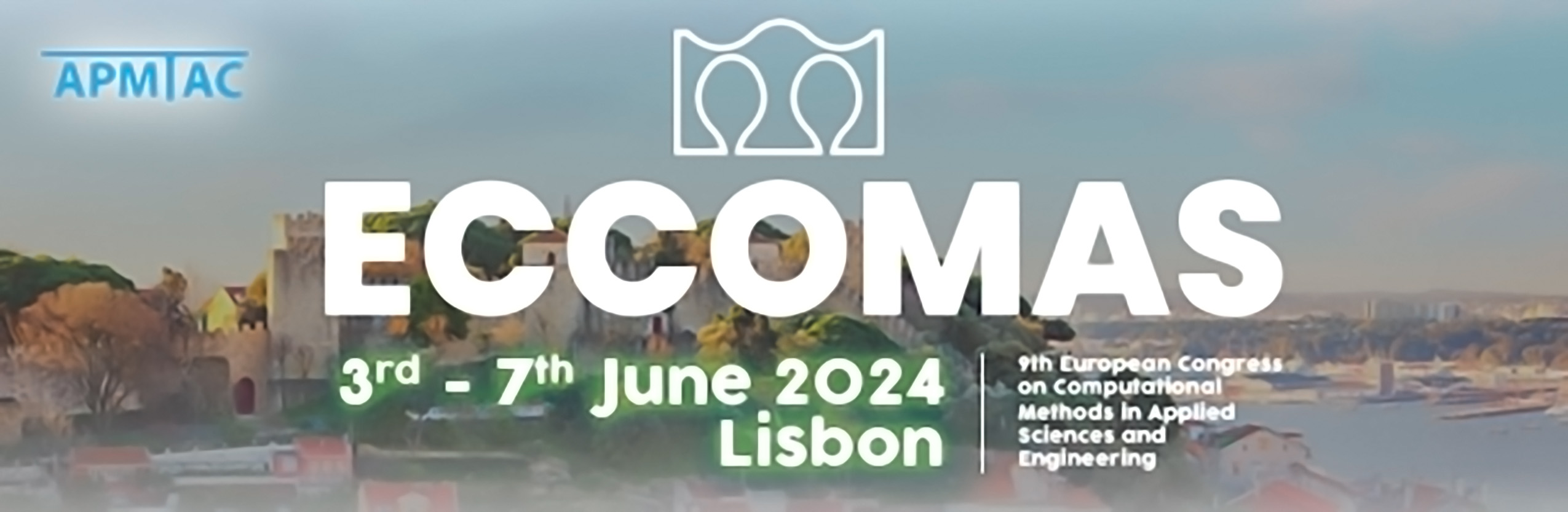
Combining Reinforcement Learning and Physics-Informed Neural Networks for Optimizing the Shape of Flow Channels in Profile Extrusion Dies
Please login to view abstract download link
Profile extrusion is a continuous manufacturing process used to produce plastic profiles with a specific cross-sectional area. Of particular interest is the design of the die through which the melt is pressed to achieve the desired shape. Due to an inhomogeneous velocity distribution at the die exit, the cross-sectional area of the produced part often deviates from the desired one. The overall goal is to prevent these deviations by computationally optimizing the shape of the die. In previous work by the authors, Reinforcement Learning (RL) has been proposed as a novel learning-based approach to tackle this design optimization problem [1,2]. RL is based on trial-and-error interactions of an agent with an environment. For each action, the agent is rewarded and informed about the subsequent state of the environment. Using RL over classical optimization approaches is justified by the fact that the agent learns a more general strategy for generating optimal shapes instead of concentrating on a single problem, which is beneficial in the design optimization of flow channels in profile extrusion dies, which often have very similar characteristics. While the authors of [1] were able to validate the approach, further investigations in [2] showed that the computation times even for simple two-dimensional test cases already exceeded multiple days. Therefore, in this talk we propose to replace the high-fidelity computational fluid dynamics simulation model by a previously trained Physics-Informed Neural Network based reduced order model as recently proposed in [3]. By combining these two scientific machine learning techniques, we expect to significantly speed up the training of the RL agent, while maintaining a similar accuracy of the predicted flow fields in the modified geometries compared to the high-fidelity approach. References: [1] D. Wolff, C. Fricke, M. Kemmerling, and S. Elgeti, “Towards shape optimization of flow channels in profile extrusion dies using reinforcement learning,” PAMM, vol. 22, no. 1, p. e202200009, 2023. [2] C. Fricke, D. Wolff, M. Kemmerling, and S. Elgeti, “Investigation of reinforcement learning for shape optimization of 2D profile extrusion die geometries,” Advances in Computational Science and Engineering, vol. 1, no. 1, pp. 1–35, Wed Mar 01 00:00:00 EST 2023. [3] S. Tillmann, D. Hilger, N. Hosters, and S. Elgeti, “Shape-optimization of extrusiondies via parameterized physics-informed neural networks,” PAMM, vol. 23, no. n/a, p. e20230