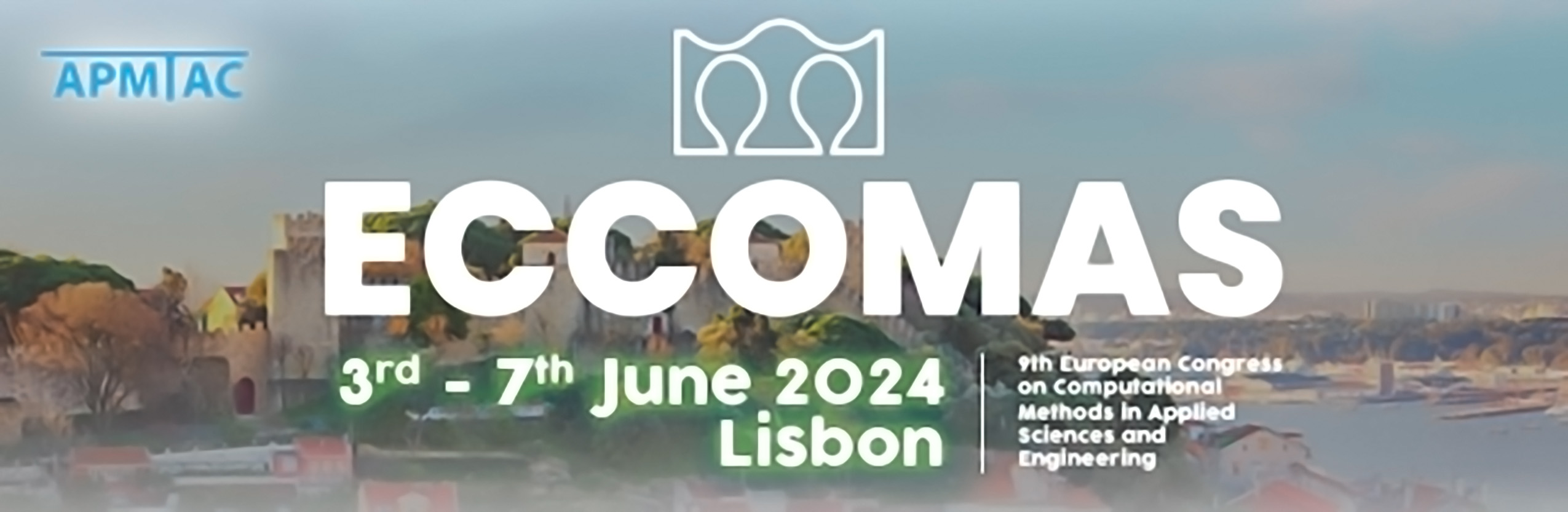
Clustered Federated Learning Through Consensus-based Optimization
Please login to view abstract download link
Federated learning is an important framework in modern machine learning that seeks to integrate the training of learning models from multiple users, each user having their own local data set. In clustered federated learning, one assumes an additional unknown group structure among users, and the goal is to train models that are useful for each group, rather than simply training a single global model for all users. In this paper, we propose a novel solution to the problem of clustered federated learning that is inspired by ideas in consensus-based optimization (CBO). Our new CBO-type method is based on a system of interacting particles that is oblivious to group memberships. Our model is motivated by rigorous mathematical reasoning, including a mean field analysis describing the large number of particles limit of our particle system, as well as convergence guarantees for the simultaneous global optimization of general non-convex objective functions in the mean-field regime. Experimental results demonstrate the efficacy of our FedCBO algorithm compared to other state-of-the-art methods and help validate our methodological and theoretical work. This is a joint work with Jose A. Carrillo, Nicolas Garcia Trillos and Sixu Li