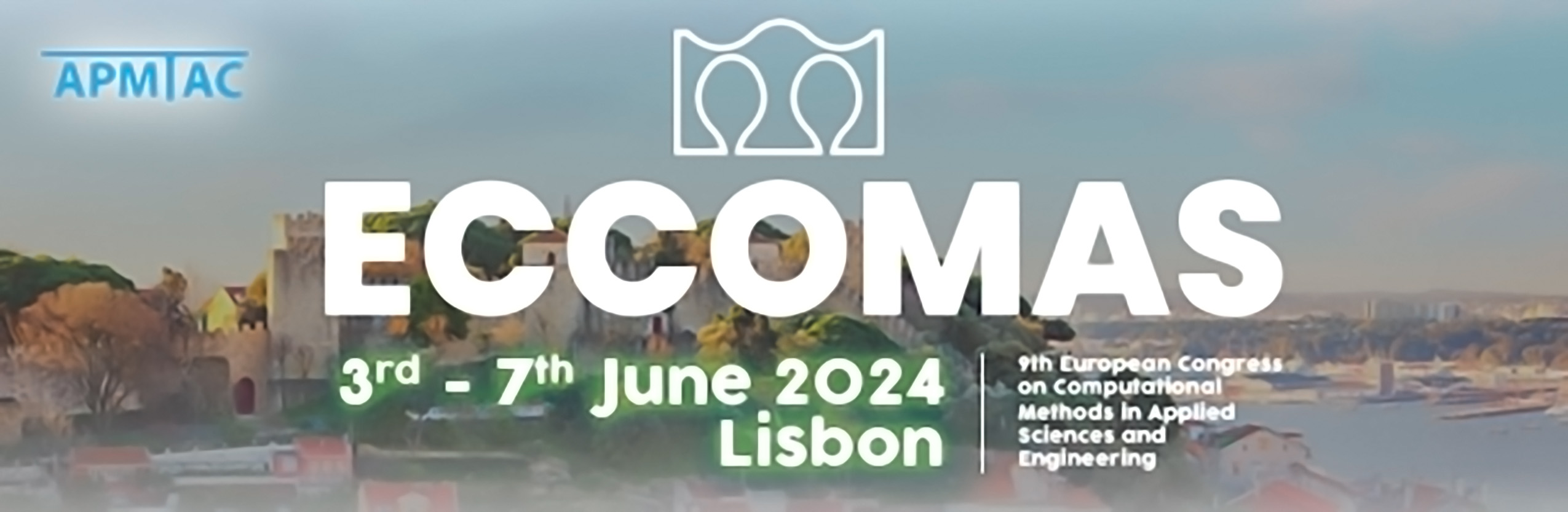
Inferring Unknown Unknowns with the Real-Rime Data Assimilation with the Regularized Bias-Aware Ensemble Kalman Filter
Please login to view abstract download link
Because of modelling assumptions, reduced-order models might be affected by state and parameter uncertainties as well as by model errors. Model errors, which are also known as biases or systematic errors, are challenging to estimate because they are ‘unknown unknowns’. With model errors, data assimilation methods are typically not well-posed because either the estimators are usually assumed unbiased (like in the Kalman filter), or they rely on some modelling assumptions on the model error. We design a real-time data assimilation framework to perform combined state, parameter, and model error estimation, i.e., the regularized bias-aware ensemble Kalman Filter (r-EnKF). We propose a reservoir computer for the model error inference. Finally, we apply the r-EnKF to synthetic data from prototypical nonlinear systems, and experimental data from hydrogen-based gas turbines. The r-EnKF successfully infers in real-time parameters and states, and the model error. The The EnKF opens new opportunities real-time assimilation in nonlinear systems.