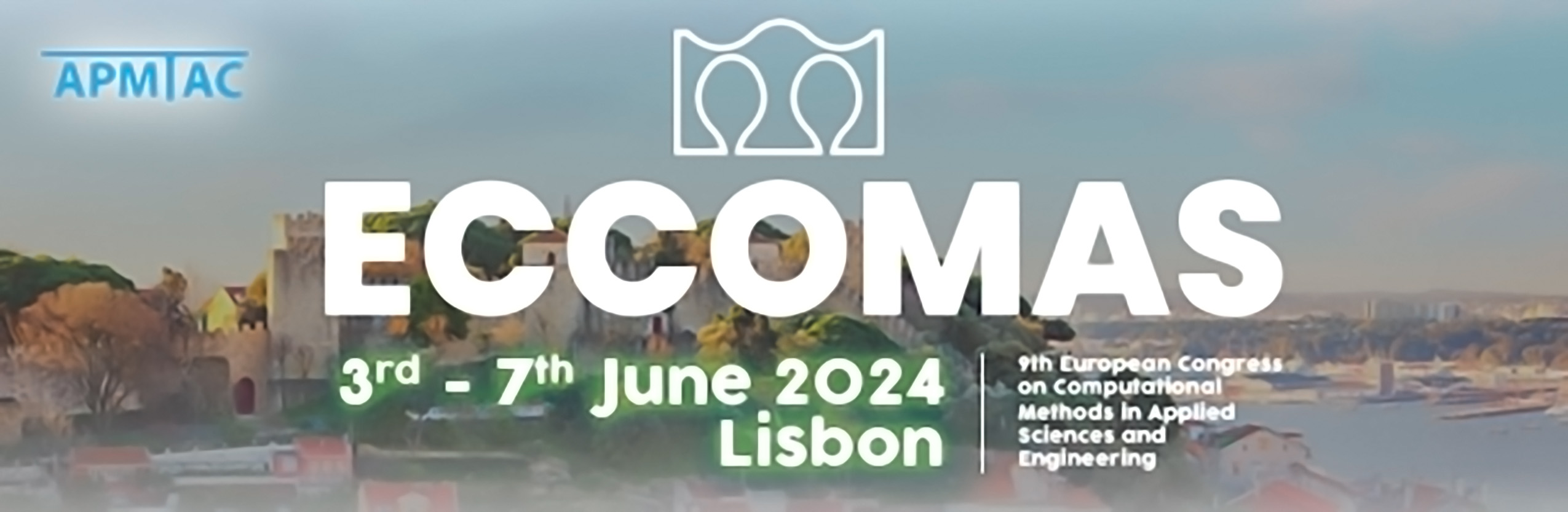
Unveiling soft biological tissues via Constitutive Artificial Neural Networks (CANNs)
Please login to view abstract download link
The classical, theory-driven approach to describe the deformation of a material body relies on the formulation of constitutive equations relating strains and stresses. A drawback of this approach are the efforts typically required to develop appropriate functional relations and identify material parameters . These efforts are not required in data-driven approaches to constitutive modeling. To combine the advantages and overcome the disadvantages of both theory- and data-driven constitutive modeling, we have developed the novel concept of Constitutive Artificial Neural Networks (CANNs). This machine learning approach to data-driven constitutive modeling does not require any major a priori assumptions about the constitutive law but yet incorporates substantial theoretical knowledge about continuum mechanics and constitutive theory. This way, CANNs are able to learn the constitutive law of a material from relatively small amounts of stress-strain data. Moreover, by their ability to incorporate also non-mechanical data, they cannot only describe the constitutive behavior of known materials but also predict the one of new materials, making them the ideal tool for computational biomechanics. Using data from mechanical tests, histological analyses and advanced imaging, this architecture is trained to predict the nonlinear macroscopic mechanical properties of e.g. arterial and brain tissue. Moreover, we demonstrate that our machine learning architecture is not limited to predictions but can also help to understand the mechanics of soft tissue. Using concepts of explainable artificial intelligence, we demonstrate that it enables the automatic, systematic and largely unbiased quantification of the importance of different microstructural features for the macroscopic mechanical properties.