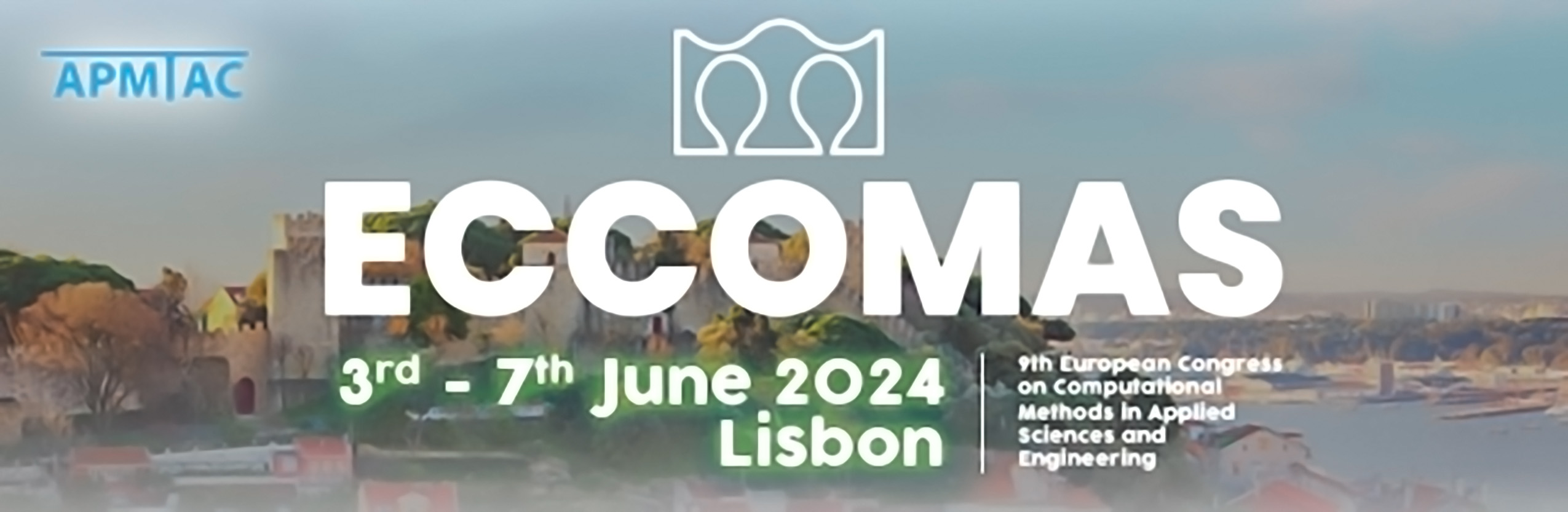
Training Deep Neural Networks Using Multilevel and Domain Decomposition Methods
Please login to view abstract download link
The training of deep neural networks (DNNs) is traditionally accomplished using stochastic gradient descent or its variants. While these methods have demonstrated certain robustness and accuracy, their convergence speed deteriorates for large-scale, highly ill-conditioned, and stiff problems, such as ones arising in scientific machine learning applications. Consequently, there is a growing interest in adopting more sophisticated training strategies that can not only accelerate convergence but may also provide parallelism, convergence control, and automatic selection of certain hyper-parameters. In this talk, we propose to enhance the training of DNNs by leveraging nonlinear multilevel and domain decomposition methods. We will discuss how to construct a multilevel hierarchy and subdomains by exploring the structure of the DNN architecture. The effectiveness of the devised training strategies will be demonstrated through a series of numerical experiments from the field of image classification, physics-informed neural networks and operator learning.