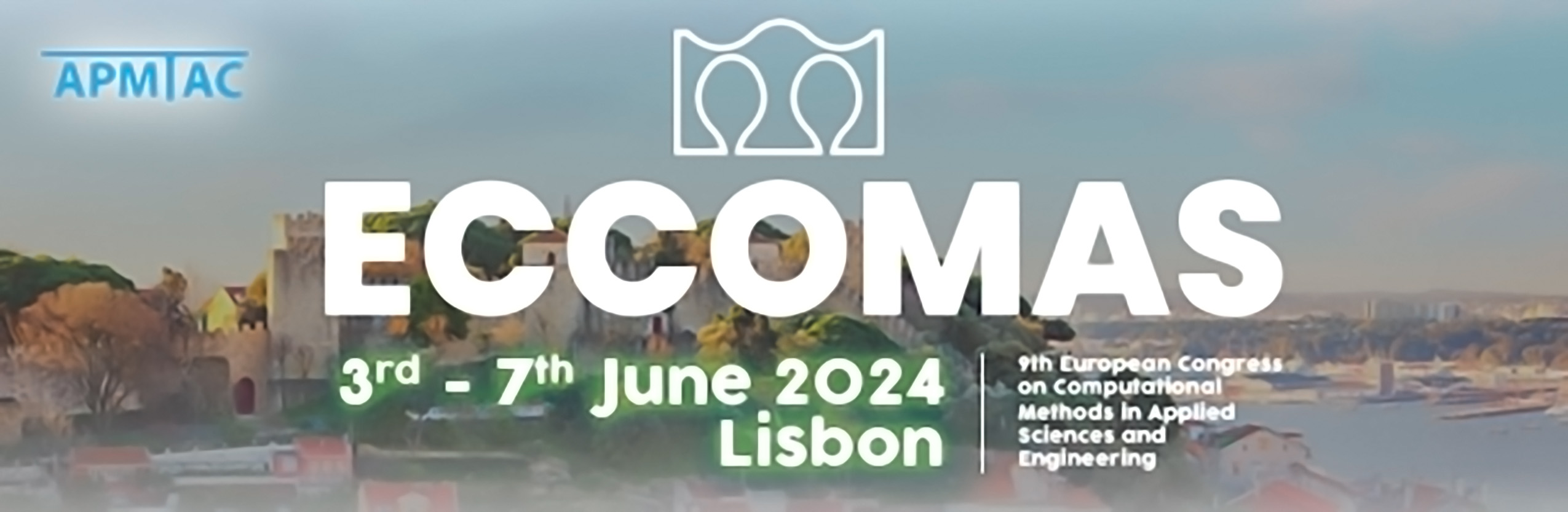
Copula-based adaptive importance sampling for sensitivity-informed reliability analysis
Please login to view abstract download link
The electricity production industry requires a high level of safety for the complex systems it operates. Assessing the reliability of such a complex system first relies on its deterministic numerical model to simulate its physical behavior. Then, the uncertainties associated with the model's inputs are often described in a probabilistic framework and propagated through the model. Finally, one can estimate a risk measure associated with the resulting random input (e.g., a rare event probability). This estimation quickly becomes challenging for highly nonlinear models and for rare events. To address this challenge, the structural reliability community has developed a variety of techniques. Adaptive importance sampling methods, such as nonparametric adaptive importance sampling (NAIS) and cross-entropy adaptive importance sampling (CE-AIS), approximate the optimal auxiliary density by an iterative process that minimizes the importance sampling variance. This mechanism significantly simplifies the calculation of rare event probabilities. However, CE-AIS lacks flexibility, while NAIS demonstrates weak performance in medium-dimensional problems. In this work, a new adaptive importance sampling based on the nonparametric Bernstein copula is proposed. The proposed method decomposes the fit of iterative conditional distributions into a fit of their marginals by univariate kernel density estimation and a fit of their copula by empirical Bernstein copula. This approach facilitates the resolution of medium-dimensional problems and is highly flexible. Additionally, this technique directly offers dedicated sensitivity information as a post-processing of the reliability analysis. Thanks to its independent sampling, a reliability-oriented sensitivity analysis is performed on each subset using sensitivity measures such as the target-HSIC (Hilbert Schmidt Independence Criterion). This additional sensitivity analysis is a simulation-free complementary tool, improving the understanding of a problem and making its resolution more robust.