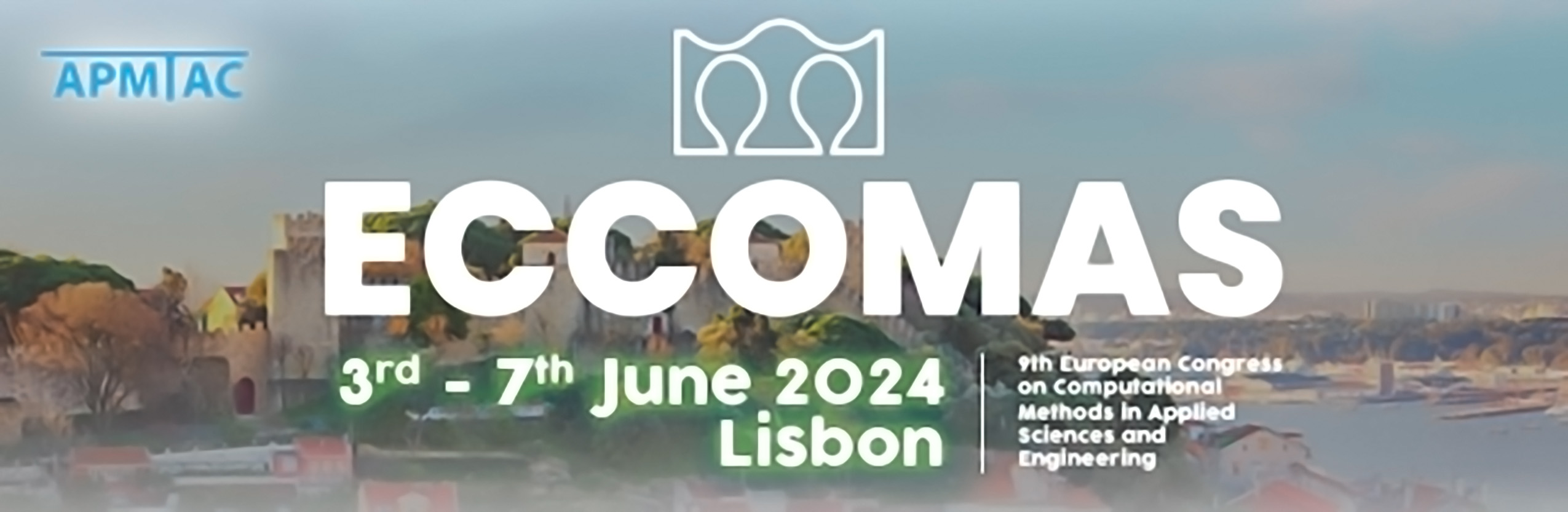
A minimally intrusive nonlinear model order reduction technique for structural mechanics
Please login to view abstract download link
Non linear simulations are challenging and computationally expensive. Reduced order modeling (ROM) reduces significantly the computational cost of such problems. However, many of ROM approaches, like Proper Generalized Decomposition (PGD) are highly intrusive and are hard to be integrated to commercial Finite Element (FE) software. The main objective of this research work is to propose a non intrusive model reduction technique for nonlinear analysis. However, ROM techniques require having in hand the stiffness matrix which evolves in time in a nonlinear problem. Therefore, our method is based on neural network training to compute our reduced stiffness matrix. In the online stage, a machine learning based regression links the weights of the reduced basis and the nodal displacements enabling real-time operations. The method is tested on linear and nonlinear cases. The results confirm its accuracy and robustness.