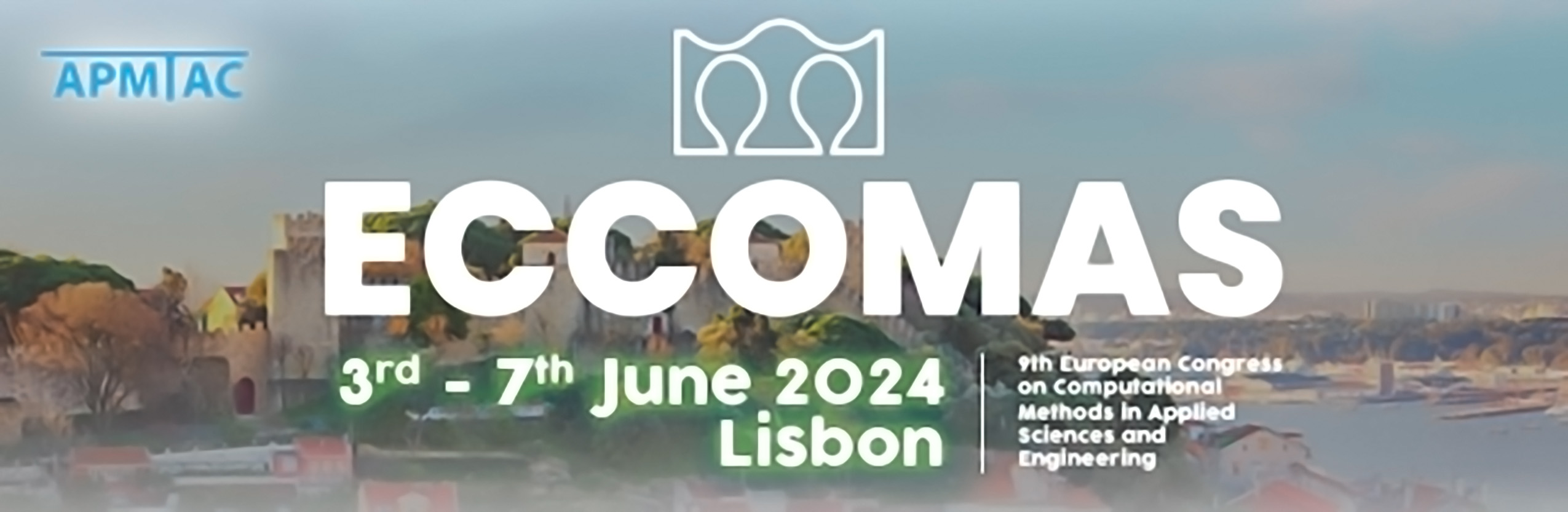
Model-based and Data-driven Digital Twins for Railway Vehicle-Track Interaction Monitoring
Please login to view abstract download link
Monitoring the vehicle-track interaction is crucial for the reliability and safety of rail transport. It is also important for the mitigation of rolling noise emissions and to sustain passenger comfort. A digital twin of the rail network that provides the actual condition of the infrastructure at each location on the one hand and the associated dynamic vehicle reactions on the other would therefore be of great value. Such a digital twin would benefit infrastructure operators and maintenance companies, but requires continuous measurement data from the entire track network. Traditional methods of acquiring measurement data involve manual devices or dedicated vehicles, both of which are time-consuming, impractical during normal operations, and consequently expensive. The use of in-service trains for data acquisition is a cost-effective alternative to conventional data acquisition. In this context, axle-box accelerometers, which measure vibrations related to the dynamic vehicle-track interaction, have proven to provide valuable information on the wheel [1] and track condition [2]. In this research we show how digital twins can effectively connect these sensor outputs to rail conditions and vice versa. Specifically, physical and data driven models are investigated that simulate the dynamic vehicle-track interaction. The data driven models can be formulated as regression task that can be trained bidirectionally. This allows estimation of rail profile conditions from sensor data and, inversely, prediction of vehicle dynamics from rail conditions. The paper provides an overview of existing model-based, data-driven approaches, exploring their combination, particularly through physics-informed neural networks. Various approaches are tested using real-world data from in-service vehicles. The findings reveal that even a small inclusion of physical information significantly enhances the predictive capabilities of data-driven machine learning methods. REFERENCES [1] B. Baasch, J. Heusel, M. H. Roth, and T. Neumann, “Train Wheel Condition Monitoring via Cepstral Analysis of Axle Box Accelerations,” Applied Sciences, vol. 11, no. 4, p. 1432, 2021, doi: 10.3390/app11041432. [2] J. Heusel, B. Baasch, W. Riedler, M. Roth, S. Shankar, and J. C. Groos, “Detecting corrugation defects in harbour railway networks using axle-box acceleration data,” insight, vol. 64, no. 7, pp. 404–410, 2022, doi: 10.1784/insi.2022.64.7.404.