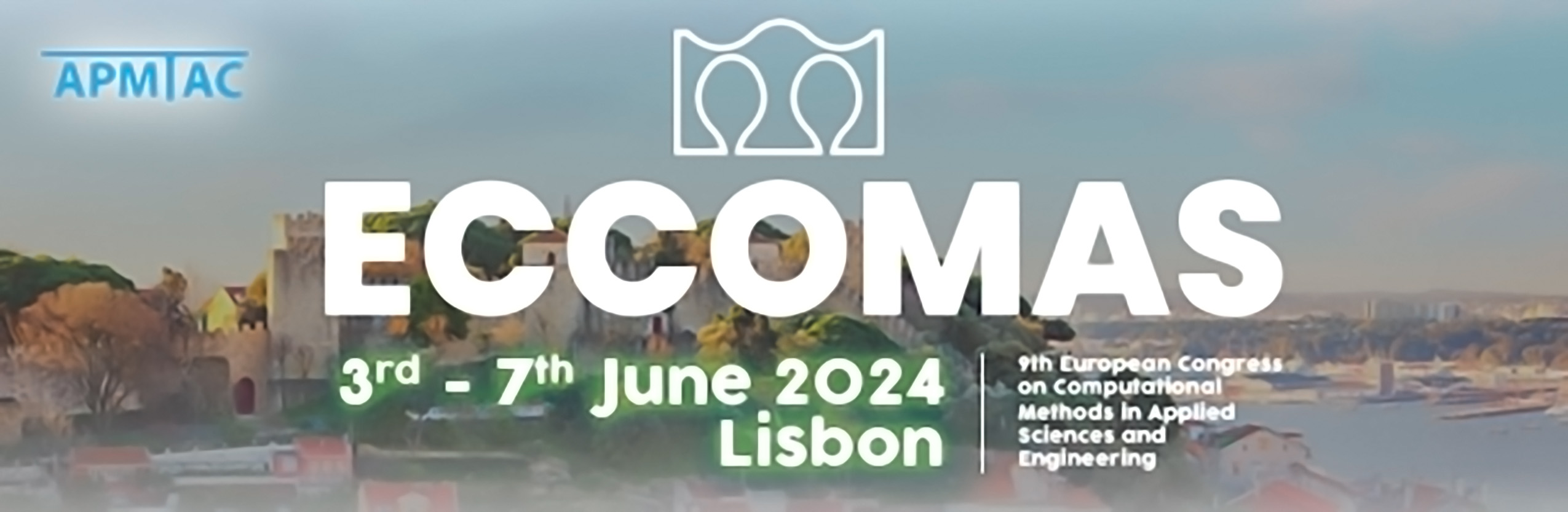
Investigating the usability of physics informed machine learning approaches for wind farm planning
Please login to view abstract download link
The uncertain behavior of wind poses a challenge towards accurately determining the wind energy yield and predicting the performance of wind farms. Multiple scenarios are required for the complex terrains to predict performance using CFD simulations (with varying grid resolutions and input parameters such as forest tree height, roughness length, wind direction, and/or thermal stability parameters). Most of these parameters vary over a wide range of time and length scales. Typically, uncertainty, variability and risk are quantified using a Monte-Carlo (MC) approach that would require a substantial number of CFD runs. However, the CFD-based simulations are computationally expensive and only a small subset of realizations can be realistically selected to do a site assessment of wind farms. The community is constantly on a lookout for improvements that could offer substantial speed up in the workflow to simulate wind farms. In this work, we investigate three variants of physics-informed machine learning approach [1] that promises substantial speed up for simulating wind farms. We set up three deep learning neural networks to learn the spatio-temporal solution of a system of partial differential equations. The networks are either data-fed and/or physics encodes employing feed forward neural network configurations. The training of the deep neural network employs physics described via partial differential equations (PDE) as rules for learning the networks. The rules also include the problems’ associated boundary and initial conditions. We investigate the feasibility of data-driven, physics-driven and a hybrid (data and physics-driven) network predictions and compare the learnt solutions against reference/numerical solutions. For each network, the data and/or physics terms are incorporated as residual terms in a loss function. A second-order L-BFGS optimization algorithm is used to minimize the loss functional to find the hyper parameters of the networks. We investigate the accuracy and bottlenecks of the trained networks by looking closely into the multi-objective loss function that is constructed by including the residual errors of the data and/or physical equations and the associated initial and boundary conditions. We present the results for RANS equations applied on a benchmark for wind farm planning.