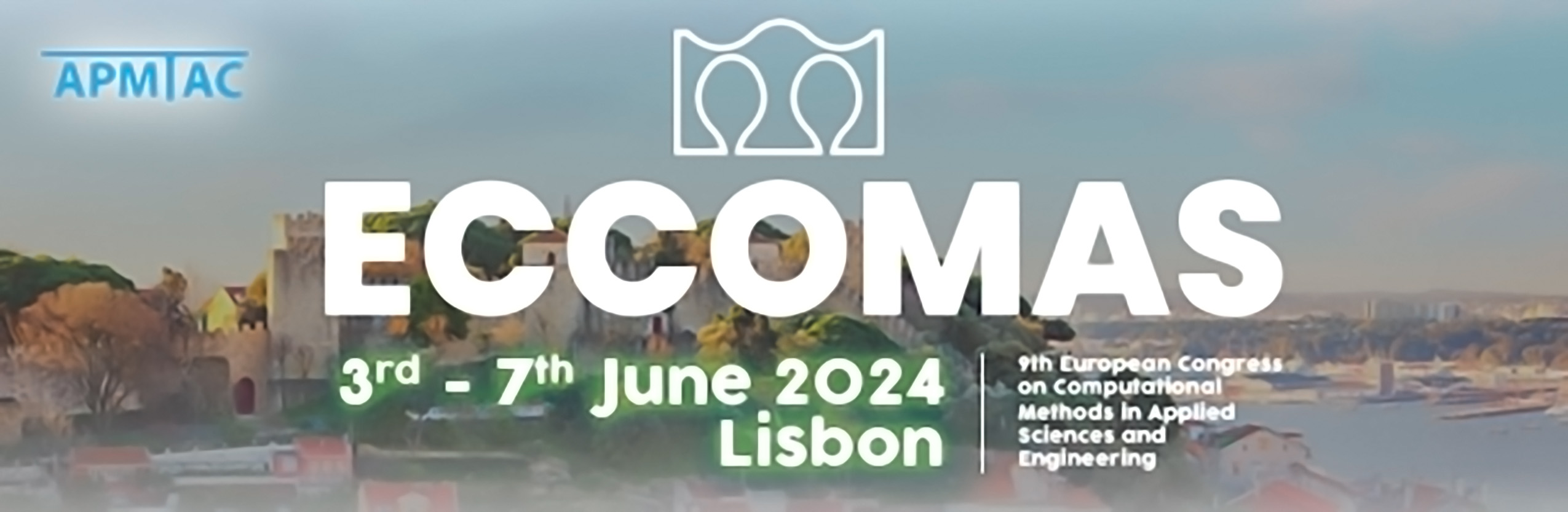
Advances in the data-driven framework to accelerate the 2-Level topology optimisation in structural problems
Please login to view abstract download link
Structural topology optimization (TO) has become a focal point in industrial design, optimizing material distribution within specified constraints. Traditional methods, such as the SIMP method, encounter challenges in achieving multi-scale structures due to strong correlations between geometric features and discretization resolution, leading to computationally intensive processes. As a result, various multi-scale techniques have emerged to reduce computational time [1]. Notably, the 2-Level TO technique proposed by [2] involves solving topological optimization in coarse discretization and subsequently addressing finer-scale TO problems in each cell, preserving structural continuity between them. However, in our increasingly demanding industrial environments, and even in certain medical applications, there is a need to further reduce computational costs. Machine learning (ML) strategies, including the one-shot approach [3], have effectively accelerated TO processes. However, these methods often encounter issues related to structural coherence, necessitating additional SIMP iterations for resolution. In response to these challenges, we introduce a data-driven framework that leverages a one-shot prediction approach within the context of 2-Level TO. Our study focuses on the critical role of defining a distance in the parameter space to minimize additional SIMP iterations. This metric serves as the foundation for defining various surrogate models and dataset creation strategies, aiming to enhance the efficiency and effectiveness of structural topology optimization.