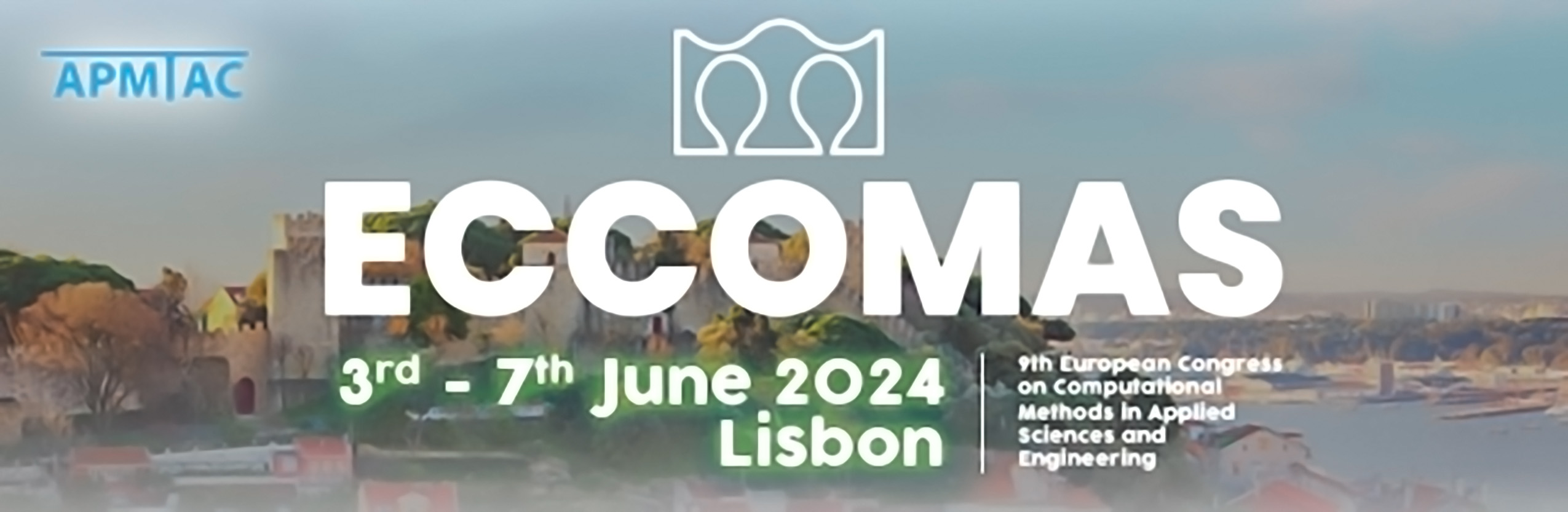
Data-driven surrogate modelling of residual stresses in Laser Powder-Bed Fusion
Please login to view abstract download link
In order to enable the industrialization of additive manufacturing, it is necessary to develop process simulation models that can rapidly predict part quality. Although multi-physics simulations have shown success at predicting residual stress, distortion, microstructure and mechanical properties of additively manufactured parts, they are generally too computationally expensive to be directly used in applications, such as optimization, controls, or digital twinning. In this talk we present a critical evaluation of how data-driven surrogate models can be used to model the residual stress of parts fabricated by Laser Powder-Bed Fusion. Three different models using varying levels of sophistication are compared: a multilayer perceptron (MLP), a convolutional neural network (CNN) based on the U-Net architecture, and an interpolation-based method based on mapping geometries onto a reference. All three methods were found to be sufficient for part design, providing mechanical predictions for a CPU time below 0.2 s, representing a runtime speed-up of at least 3900 × . Neural network-based models are significantly more expensive to train compared to using interpolation. However, the generality of models based on the U-Net architecture is attractive for applications in optimization.