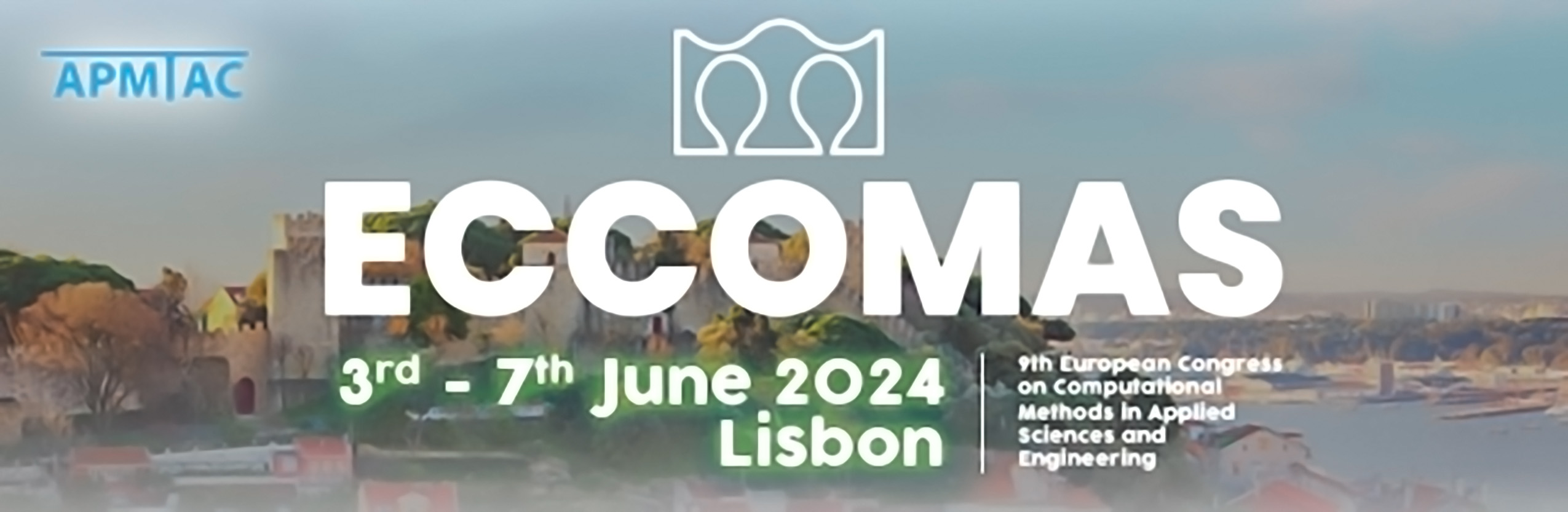
Examining the Influence of Dependent Parameters on Biomechanical Models with Global Sensitivity Analysis
Please login to view abstract download link
Predictions of biomechanical models contain significant uncertainty resulting, among others, from a lack of knowledge about their parameters. Given the impracticality of obtaining patient-specific measurements for all parameters, it is essential to prioritise identification efforts on key parameters contributing predominantly to the predictive uncertainty. Our recent research highlights the essential role of variance-based global sensitivity analysis, utilising Sobol indices, in identifying influential and uninfluential parameters within complex biomechanical models [1, 2]. However, the method assumes parameter independence, which is a notable limitation because such parameter independence does not hold for many biomechanical models. In response, we demonstrate a global sensitivity analysis method that overcomes this restriction [3]. We showcase the method’s properties and capabilities through examples, illustrating its ability to quantify the impacts of potentially dependent parameters on the predictive uncertainty of in silico biomechanical models. In conclusion, the framework presented in this study provides a reliable tool that broadens the application of global sensitivity analysis to a large class of biomechanical models, especially those featuring dependent input parameters. REFERENCES [1] S. Brandstaeter, S.L. Fuchs, J. Biehler, R.C. Aydin, W.A. Wall, C.J. Cyron. Global Sensitivity Analysis of a Homogenized Constrained Mixture Model of Arterial Growth and Remodeling. J. Elast., 145(1–2), pp. 191–221, 2021. doi: 10.1007/s10659-021-09833-9 [2] B. Wirthl, S. Brandstaeter, J. Nitzler, B.A. Schrefler, W.A. Wall. Global Sensitivity Analysis Based on Gaussian-Process Metamodelling for Complex Biomechanical Problems. Int. J. Numer. Meth. Biomed. Eng., 39 (3): e3675, 2023. doi: 10.1002/cnm.3675. [3] I. Bertrand, C. Prieur. Shapley Effects for Sensitivity Analysis with Correlated Inputs: Comparisons with Sobol’ Indices, Numerical Estimation and Applications. Int. J. Uncert. Quantif., 9 (5): 493–514, 2019. doi: 10.1615/Int.J.UncertaintyQuantification.2019028372.