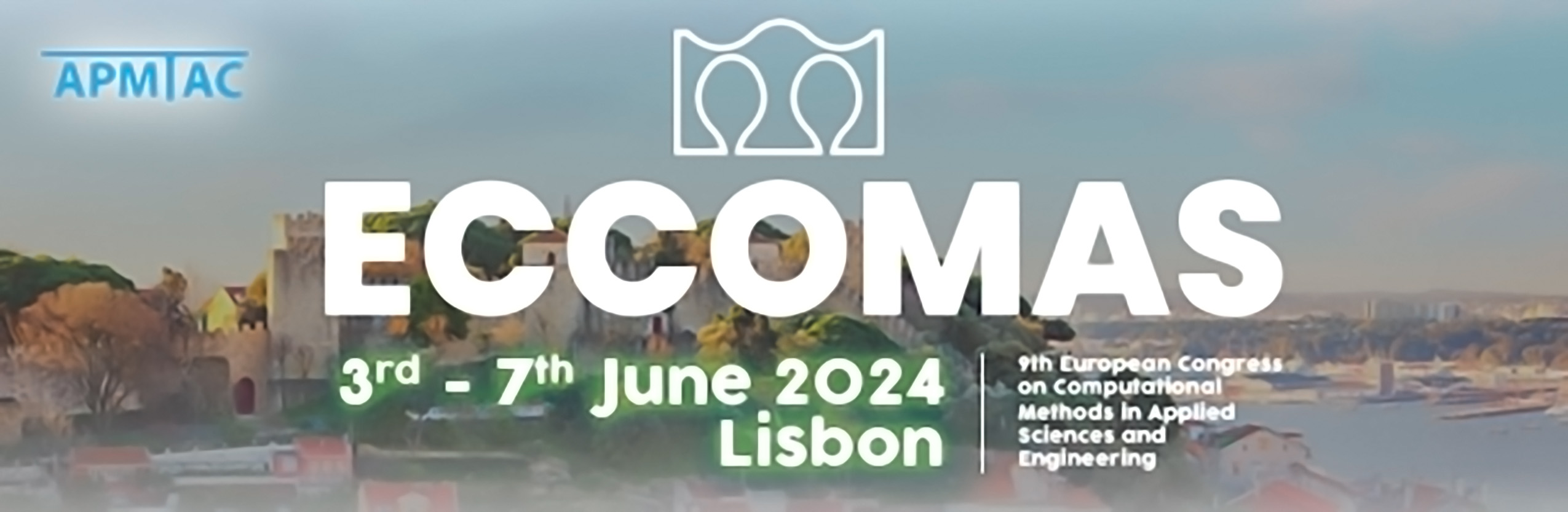
Matryoshka policy gradient for control problems
Please login to view abstract download link
Policy gradient methods in Reinforcement Learning have been very successful in solving complex tasks over the past years, dealing well with large (possibly infinite) state and action spaces. However, policy gradient methods often lack theoretical guarantees, or those obtained assume quite idealised settings, for example, finite state and action spaces. The case of infinite (continuous) state and action spaces remains mostly unsolved. In this talk, I will first introduce the Matryoshka Policy Gradient (MPG) method [1], a novel entropy regularised policy gradient algorithm for finite horizon tasks. It uses softmax policies and relies on the following idea: by fixing in advance a maximal horizon N , the agent trained with MPG learns to optimize policies for all smaller horizons simultaneously, that is from 1 to N , in a nested way (hence the name from Matryoshka dolls). Under mild assumptions, we prove uniqueness and characterize the optimal policy, together with global convergence of MPG. Most notably, these results hold for infinite continuous state and action spaces. Then, I will talk about recent extensions of the MPG method to tackle optimal control tasks governed by ODEs. We evaluate modifications to the MPG algorithm, namely, a PPO-like update [2] and an actor-critic formulation [3] in order to understand its impact in the robustness of solving optimal control tasks.