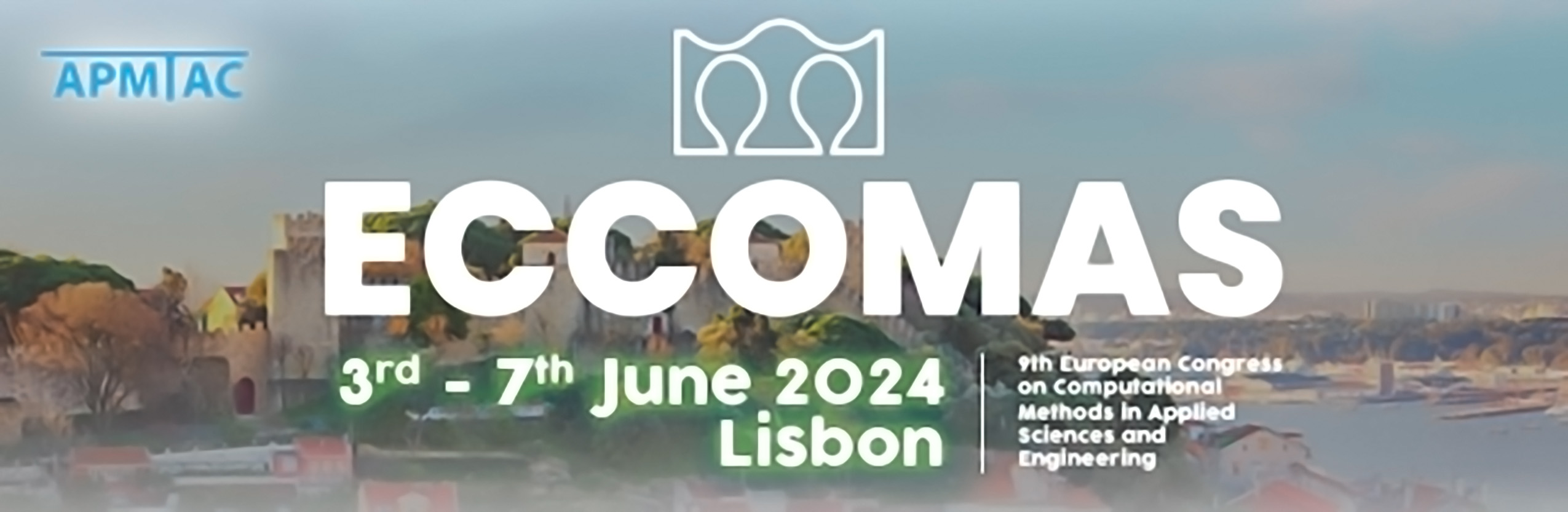
Enhancing Thermal Conductivity Modeling of Polyurethane with Phase Change Materials via Physics-Informed Neural Networks at Multiple Scales
Please login to view abstract download link
Polyurethane (PU) boasts exceptional thermal properties, making it an attractive choice for thermal insulation. The incorporation of Phase Change Materials (PCMs) capsules into Polyurethane (PU) has emerged as a highly effective method for enhancing building envelope performance. This innovative configuration significantly enhances indoor thermal stability while reducing fluctuations in indoor air temperatures. To investigate the thermal conductivity of the PU-PCM composite material, we propose the use of a hierarchical multi-scale model that employs Physics-Informed Neural Networks (PINNs). This model allows for precise prediction and analysis of the material's thermal conductivity at both micro and macro scales. By harnessing the synergy between physics-based knowledge and data-driven learning inherent in PINNs, we adeptly address complex inverse problems and multi-scale phenomena. Furthermore, the insights gained into thermal conductivity facilitate material design optimization. To comprehensively consider occupants' thermal comfort within a building enclosure, we conduct a case study to evaluate the performance of this optimized material in a single-room setting. Simultaneously, we estimate the energy consumption associated with this scenario. All findings collectively highlight the promising potential of this design, enabling passive building energy management and significantly improving occupants' comfort. The successful development of this multi-scale model based on PINNs holds immense promise for advancing our understanding of PU-PCM's thermal properties. It is positioned to make substantial contributions to the formulation and enhancement of materials for various practical applications, including thermal energy storage systems and the design of insulation for cutting-edge building envelopes.