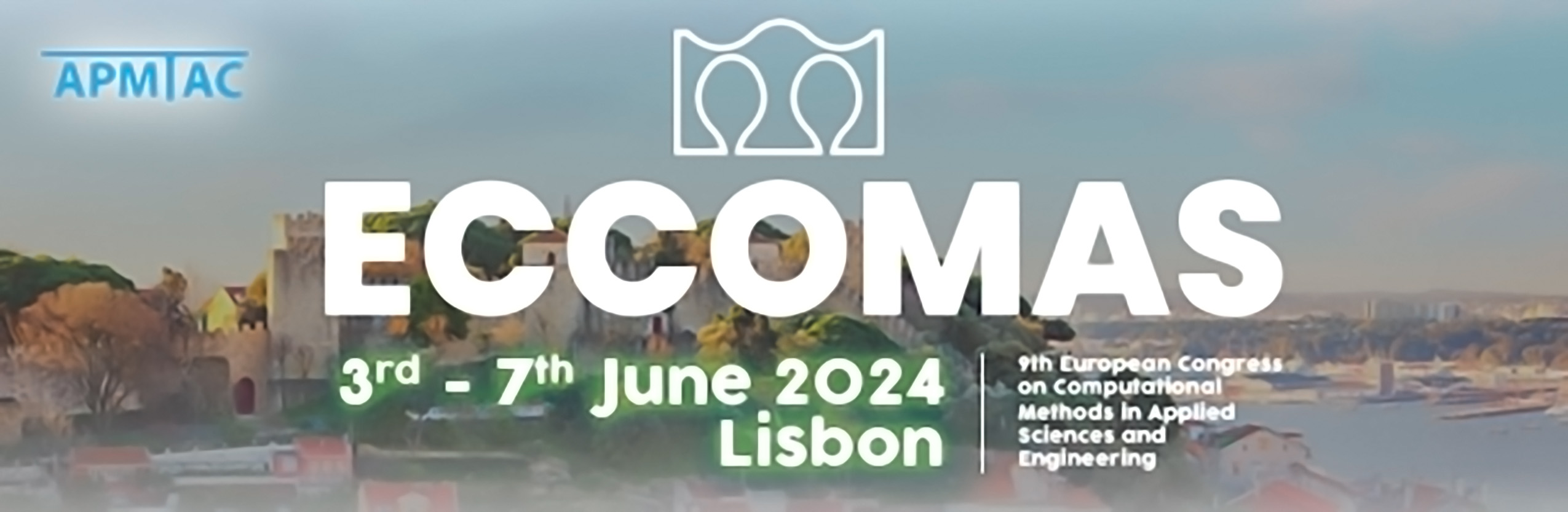
Learning Discretization Invariant Closure Models for Large Eddy Simulation of Turbulent Flows
Please login to view abstract download link
The incompressible Navier-Stokes equations form a model for the evolution of fluid flows. For turbulent flows, solving these equations numerically can be computationally expensive. Large Eddy Simulation (LES) aims to resolve the large scales only, which allows for using coarser computational grids. This is made possible by using a closure model to account for the effect of the unresolved scales. Traditionally, LES closure models have been formulated in the continuous setting, with very few model parameters. Recently, neural networks have been used to learn discrete closure models, but these do not naturally generalize to other computational grids than the one trained for. Neural operators address this problem by parameterizing continuous operators instead of discrete ones. In a recent work, we showed that discretizing the equations first, before filtering out the small scales, provides an exact framework for learning discrete closure models for simple model equations. We have since extended this framework to the incompressible Navier-Stokes equations, using a family of discrete filters that preserves incompressibility. We compare Fourier neural operators, discretization informed convolutional neural networks, and traditional closure models on seen and unseen grids. The results show that performant discretization invariant closure models can be learned for filtered turbulent flows, while enforcing incompressibility by design.