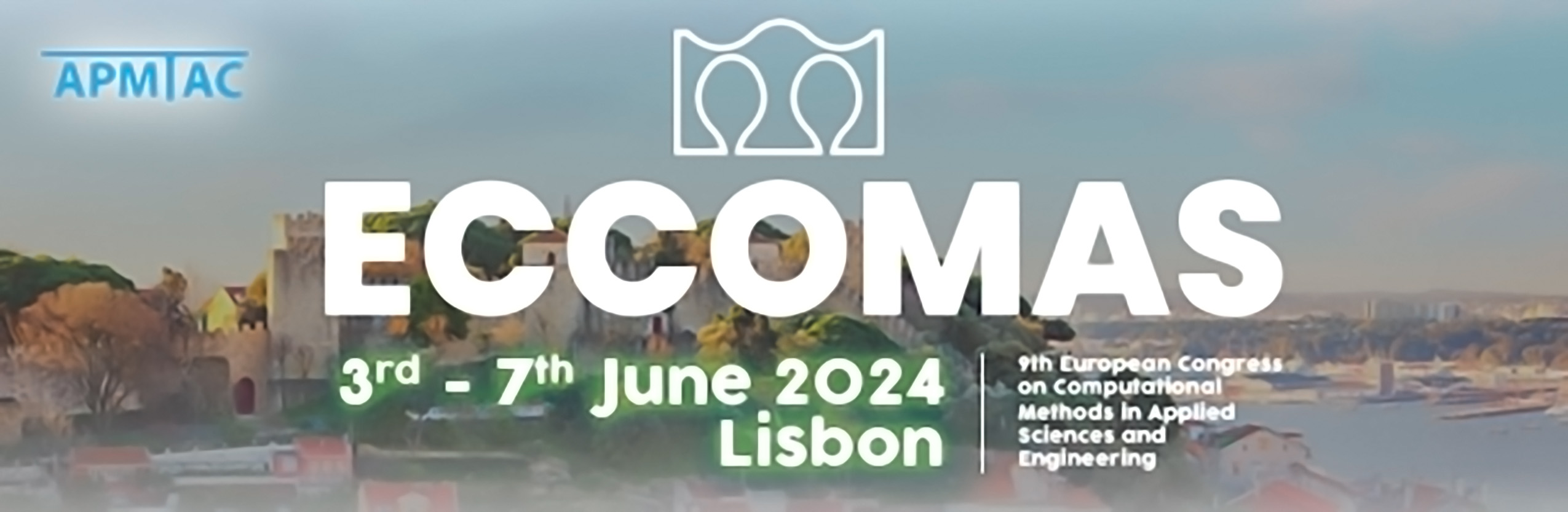
Multi-fidelity sampling uncertainty quantification: overview, recent trends, and perspectives
Please login to view abstract download link
Uncertainty Quantification (UQ) is a key component of the Verification and Validation paradigm that allows for predictive science with computer models. The last two decades saw a maturation of algorithms and software for UQ, however, little attention has been devoted to the use of UQ for high-fidelity models. Since both the computational cost and the accuracy of UQ methods scale with the available number of simulations, performing UQ for applications involving limited computational resources remains prohibitive. Multi-fidelity UQ emerged as a possible strategy to overcome this challenge. The idea is simple and effective: complement a limited number of high-fidelity runs with a larger dataset based on low-fidelity evaluations. Moreover, for applications involving high- dimensional parameter inputs and poor solution regularity, multi-fidelity sampling approaches are often the method of choice. For this class of methods, the idea is to leverage the correlation among information sources such that an optimal allocation problem can be solved to determine the number of evaluations on each model under a prescribed budget. In this talk we will provide an overview of multi-fidelity sampling strategies and how improvements, to the basic formulation, can be adopted to adapt these methods to realistic applications including, but not limited to, non-hierarchical ensembles of models [1], low-fidelity hyperparameters tuning, dissimilar parametrization [2], stochastic solvers [3], etc. We will discuss both recent trends and current challenges that we will need to address to even improve the current state-of-the-art. REFERENCES [1] A.A. Gorodetsky, G. Geraci, M.S. Eldred, and J.D. Jakeman, A generalized approxi- mate control variate framework for multifidelity uncertainty quantification, J Comp. Physics, 408, 109257, 2020. [2] X. Zeng, G. Geraci, M.S. Eldred, J.D. Jakeman, A.A. Gorodetsky, R. Ghanem Multi- fidelity uncertainty quantification with models based on dissimilar parameters, Comp. Meth. App. Mech. and Eng., 415, 116205, 2023. [3] B.W. Reuter, G. Geraci, and T.M. Wildey, On the challenges of sampling-based multifidelity uncertainty quantification for non-deterministic models, Int. J. for Unc. Quant., Under review, 2024. SNL is managed and operated by NTESS under DOE NNSA contract DE-NA0003525.