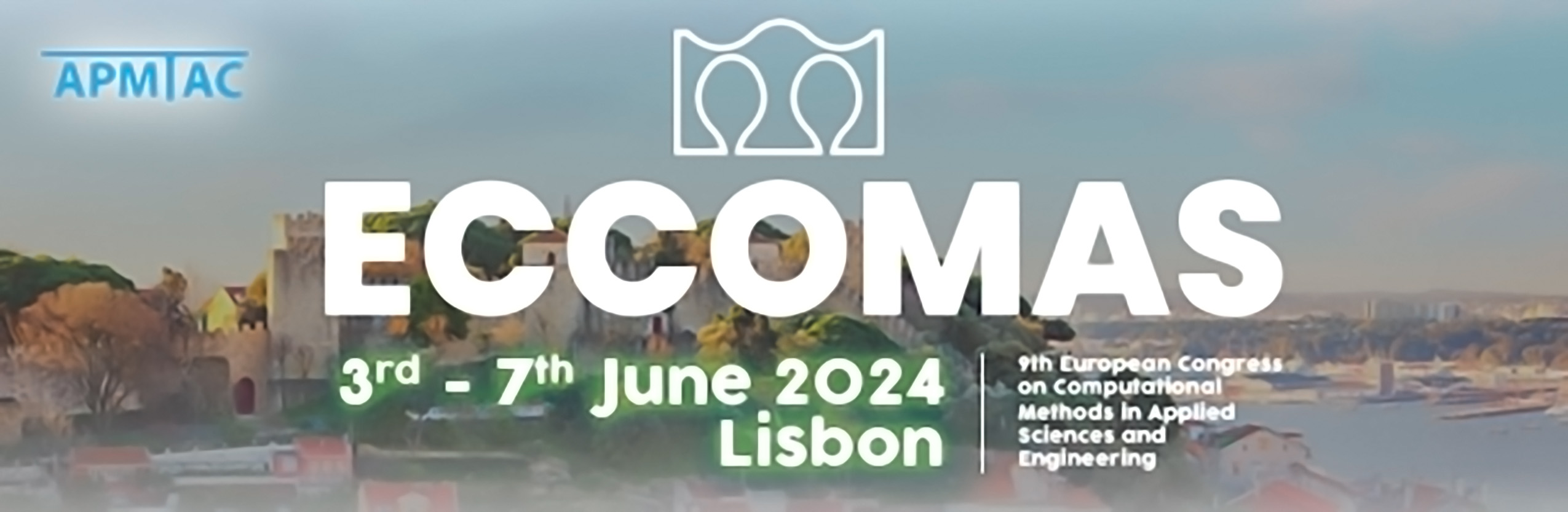
Data-Centric Approaches for Adaptive Vibration Control Systems Incorporating Superelastic Shape Memory Alloys
Please login to view abstract download link
Due to their superb hysteretic energy dissipation and deformation capabilities, superelastic shape memory alloy (SMA) wires and bars are increasingly integrated into vibration control devices of civil engineering structures. Transformation between two solid phases, austenite and martensite, allows the SMAs to sustain strains greater than 8%, offering excellent ductility, damping properties, and fatigue resistance. These unique properties open up innovative solutions to improve resilience and adaptability in structures. In particular, for the design of adaptive vibration control strategies that incorporate SMAs, accurate and efficient material models are required. However, the response of superelastic SMAs, particularly under dynamic loading, is highly influenced by complex thermodynamic effects. The precise representation of these effects in constitutive models poses a significant challenge, which requires an accurate identification of the model parameters and variables. In the context of vibration control, martensite volume fraction is an important indicator of the level of energy dissipation of SMAs. Close to 100% martensite, SMAs can efficiently dissipate vibration energy. However, when martensite evolution is complete, additional loading could cause damage. Therefore, to avoid damage and achieve optimal usage, we present a deep operator network-based (DeepONet) approach that estimates the evolution of the martensite volume in SMAs directly from strain time histories. Based on the proposed martensite estimation method, our study shows that vibration control devices can be easily assessed. During training, the DeepONet is constraint by a martensite-dependent stress equation such that only stress-strain data are required for training. After training, the stress response can also be obtained from the output of the DeepONet by using the same equation. Furthermore, to reduce the experimental effort and enhance the generalizability of the model, we show how to include also synthetic data for the training process. Thus, a constitutive macroscopic material model is used, in which model parameters are tuned using a further approach based on a feedforward neural network. This method only requires a small set of cyclic tensile tests to accurately map the stress-strain behavior of SMAs. The effectiveness of the proposed methods is demonstrated through stress-strain experiments conducted on superelastic SMA wires.