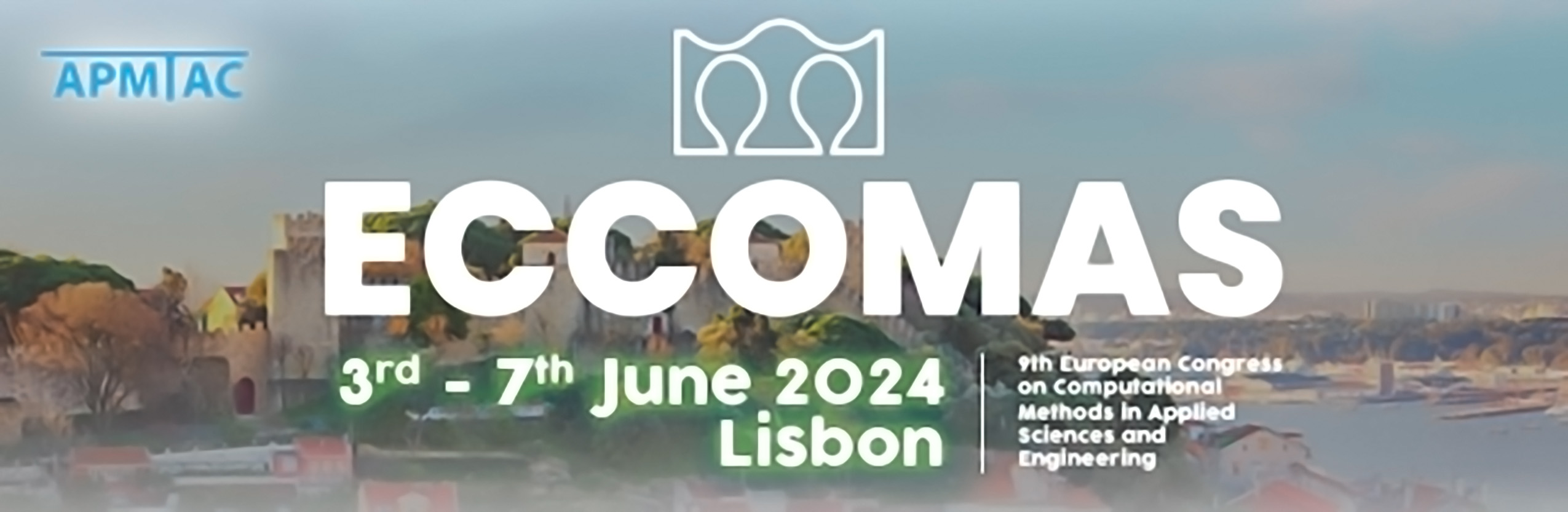
Randomized neural network with Petrov–Galerkin methods for solving nonlinear PDEs
Please login to view abstract download link
We propose a novel approach for solving partial differential equations (PDEs) based on randomized neural networks and the Petrov–Galerkin method, which we name the RNN-PG methods. This approach employs randomized neural networks to approximate unknown functions and enables a flexible selection of test functions, such as finite element basis functions, Legendre or Chebyshev polynomials, or neural networks. We use the RNN-PG methods to solve nonlinear PDEs, such as Burger’s equations and Navier-Stokes equations. Numerical experiments demonstrate that the RNN-PG methods can attain high accuracy with a small number of degrees of freedom. Furthermore, RNN-PG has several benefits, such as being mesh-free, handling different boundary conditions easily, and solving time-dependent problems efficiently by using a space-time approach. These results reveal the great potential of the RNN-PG methods in the field of numerical methods for PDEs.