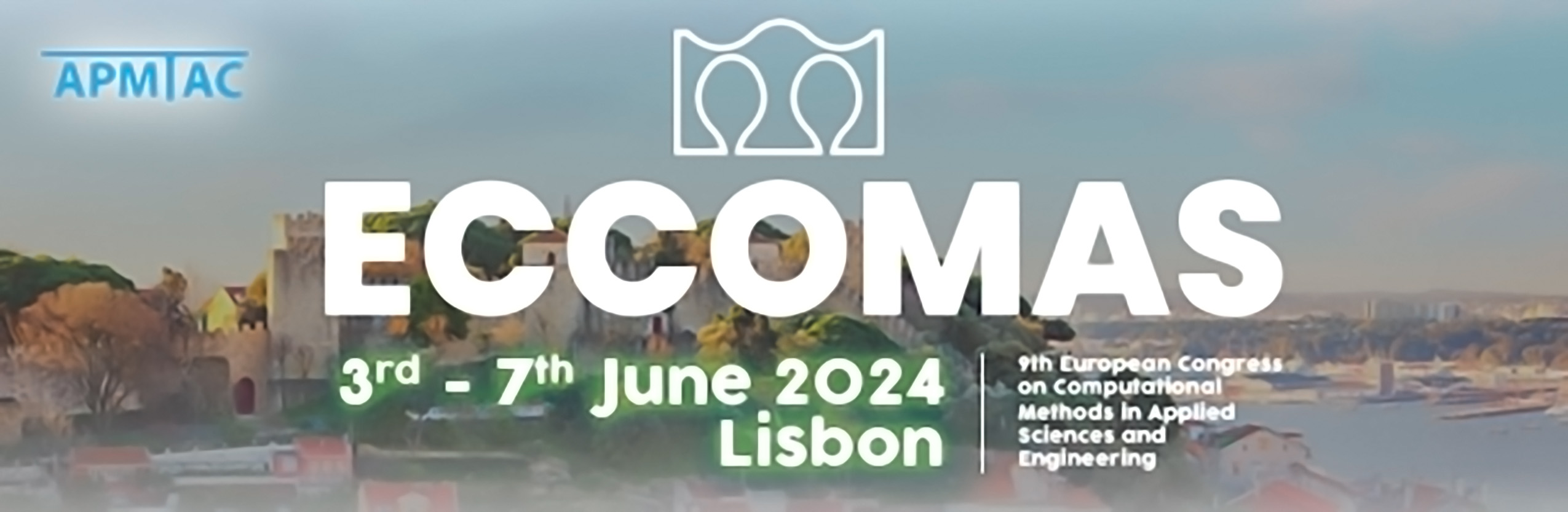
Mixture Density Network for the Prediction of the Wall Shear Stress Statistical Moments for Turbulent Separated Flows
Please login to view abstract download link
In turbomachinery, boundary layers on compressor and turbine blades may separate due to adverse pressure gradients and curvature effects, affecting the machine's performance. Engineers are interested in accurately predicting of this phenomenon. Direct Numerical Simulations of turbomachinery flows are still intractable, even on modern supercomputers. Wall models have been developed to significantly reduce the computational cost associated with resolving the boundary layer. Considerable efforts are being made to improve wall models to make them more robust and applicable to a wide range of flow conditions. Even Machine Learning and Deep Learning techniques have also emerged to support the development of new turbulence models. Moreover, DL techniques offer the opportunity to avoid making assumptions about the underlying physics. In this context, neural networks are trained to develop new wall shear stress (WSS) models for separated~flows. The present work uses a specific network to predict the WSS probability density function (PDF) without making any prior assumptions (i.e., fully turbulent, at equilibrium, and attached boundary layer). The data-driven WSS model takes as input a stencil of ten quantities (velocity, pressure gradients, curvature). The model outputs the PDF of the wall-parallel components of the WSS. The model is trained on a database combining sufficiently complex physics with a number of test cases, including turbulent channel flows, the two-dimensional periodic hill, and a low-pressure compressor (LPC) cascade. The periodic hill presents a massive separation from the hill crest and the LPC cascade, an incipient separation near the trailing edge on the suction side. The database is non-dimensionalized with the viscosity and the wall-model height for better generalizability. The model is evaluated a posteriori by performing wmLES using the in-house flow solver Argo-DG on two channel flows and one separated flow. The MDN model, applied to the turbulent channels, outperforms the standard and other recently developed data-driven WSS models. The model shows a real improvement in the WSS prediction on the two-dimensional periodic hill compared to the WSS model based on Reichardt's velocity profile, except that the reattachment location is slightly underestimated. The application of the novel model to an LPC cascade presenting a massive separation is targeted.