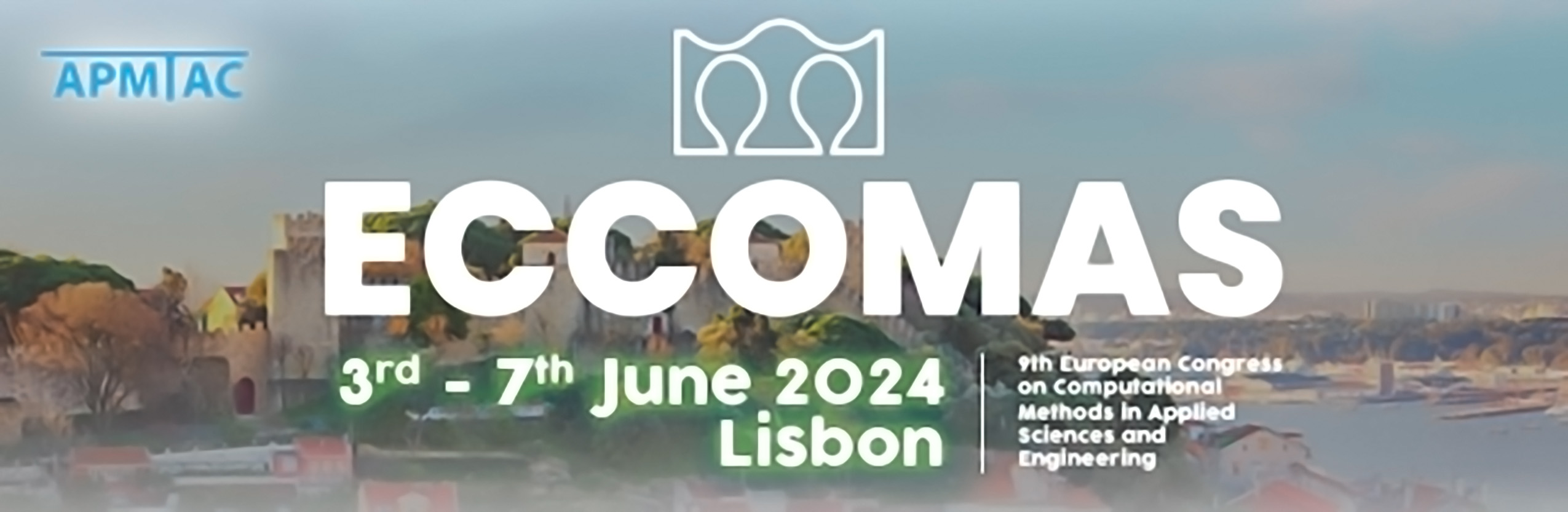
Material Design Driven by Morphology Characteristics
Please login to view abstract download link
The importance of morphology in heterogeneous material performance is well known. Setting up an inverse problem of finding suitable morphological parameters, given a known performance criterion, requires prior knowledge of the entire parameter space. However, exploring the vast parameter space of heterogeneous materials remains a major hurdle in designing high-performance materials tailored to specific applications. Experiments are expensive and time-consuming, while simulation tools use approximations of morphological features and material models. Hence, the challenge in modeling heterogeneous materials is two-fold: first, accurate computational domain representation for every possible morphology, and second, model learning rather than model approximation and fitting. Recent advancements in accurate morphology generation, employing tools like deep neural networks (DNNs), have demonstrated promising results in tackling the first challenge, although, only checking for statistical similarity of morphologies to real samples retrospectively. While, emerging tools like graph neural networks (GNNs) are still in their early stages of addressing the second challenge. Nonetheless, interpreting the parameters within a very high-dimensional latent space remains a challenge in both cases. In our research, the digital material twin is envisioned as a condensed representation of material information capable of economically addressing the inverse problem mentioned above. To this end, we focus on developing a physical parameter-driven morphology quantification and in-silico computational domain generation tool that enforces statistical similarity of morphology during the generation process. Additionally physical parameters allow for sensitivity analysis and design of experiments, which are essential elements of inverse analysis procedure. This work is performed to address gaps in experimental data, specifically focusing on structural concrete.