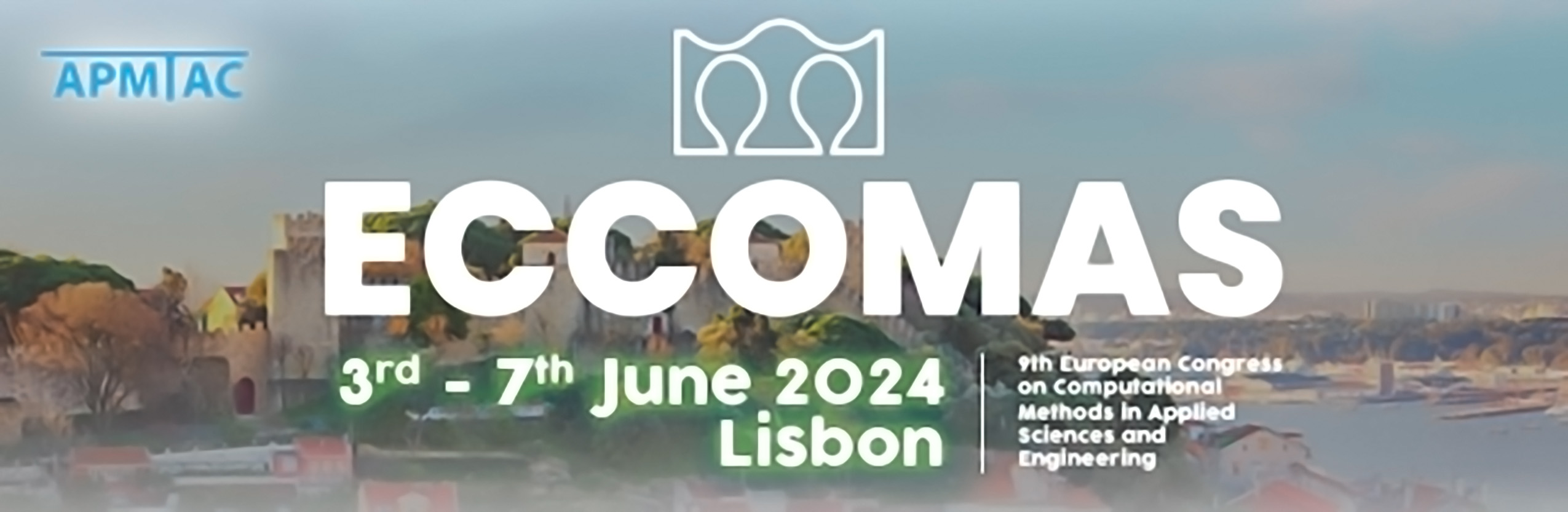
A PDE-informed and Physics-informed neural network for learning weak solutions in two-dimensional conservation laws
Please login to view abstract download link
Here we propose a hybrid PINN model [1] to learn weak solutions of conservation laws. The entropy flux, which satisfies admissibility condition and, as a consequence, grants uniqueness to the weak solution, is learned by a fully-connected neural network. The entropy rate principle as described in [2] is used to compose the PINN residual in our model. The proposed approach is also extended to problems in the two-dimensional domain as in [3]. Considering that ensuring convexity of a deep neural network output is in general not possible, here we combine the learned function with Kruzkhov entropy, as used in [4]. By setting appropriate weights to the Kruzkhov and the learned residuals, we ensure a valid weak solution under the admissibility criteria at the same time that the learning process of the entropy provides more flexibility for the model optimizer to search for the most accurate solution, i.e., weak PDE-informed. Preliminary results suggest that our model is capable of reducing the approximation error with fewer training epochs. For the inviscid Burgers' equation with rarefaction waves in 1D, x in [-1,1] and t in {0s,0.2s,0.4s}, and 100 epochs, our method achieves a relative error test of 0.105, whereas the method in [4] achieves 0.118. At the ECCOMAS 2022 [3], we have made some progress in the development of a PINN computational study for a scalar 2D inviscid Burgers model with Riemann data. We expect to improve 1D/2D weak PDE-informed and Physics-informed neural network for learning weak solutions for two-dimensional conservation laws to be presented at ECCOMAS 2024. REFERENCES [1] M. Raissi, P. Perdikaris, G. E. Karniadakis. Physics-informed neural networks: A deep learning framework for solving forward and inverse problems involving nonlinear partial differential equations. Journal of Computational Physics 378 (2019) 686-707. [2] C. M. Dafermos. A variational approach to the Riemann problem for hyperbolic conservation laws. Discrete Contin. Dyn. Syst, 23 (2009), 185-195. [3] R. Carniello, J. Florindo, E. Abreu, A PINN computational study for a scalar 2D inviscid Burgers model with Riemann data. In: ECCOMAS2022. https://www.scipedia.com/public/Carniello et al 2022a (2022). [4] T. De Ryck, S. Mishra, R. Molinaro. wPINNs: Weak physics informed neural networks for approximating entropy solutions of hyperbolic conservation laws. arXiv preprint arXiv:2207.08483 (2022).