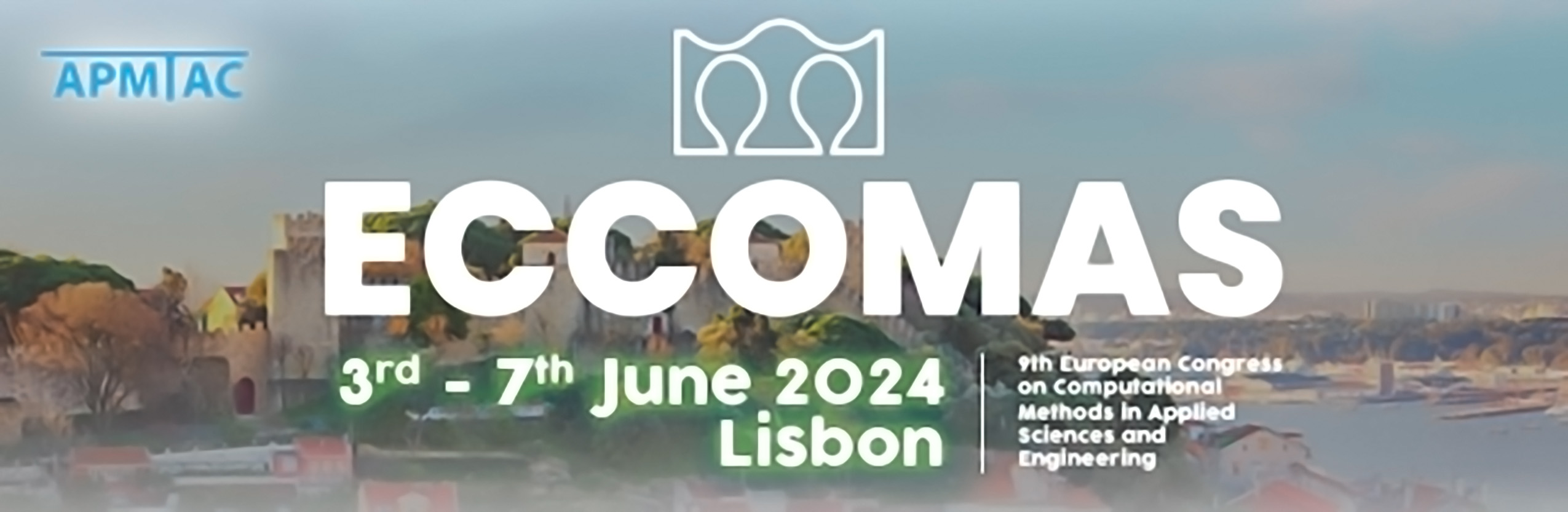
Neural Galerkin Schemes That Can Preserve Hamiltonians and Other Quantities
Please login to view abstract download link
Neural Galerkin schemes, based on the Dirac-Frenkel variational principle, train nonlinear parametrizations sequentially in time to approximate solution fields of time-dependent partial differential equations. Due to the nonlinearity of the parametrizations, few parameters efficiently capture even complex dynamics in first-principles-based physical models. Such models are often constructed based on the observation that quantities like energy, mass, and momentum remain constant over time, representing conserved quantities of the physical system. In this talk, we demonstrate how we can modify Neural Galerkin schemes such that these quantities are also conserved quantities in the approximate solutions produced by Neural Galerkin schemes. We first show that only adding constraints that aim to conserve quantities in continuous time can be insufficient because the nonlinear dependence on the parameters implies that even quantities that are linear in the solution fields become nonlinear in the parameters and are thus not conserved by standard Runge-Kutta time integration schemes. Instead, we propose Neural Galerkin schemes that compute an embedding onto the manifold of nonlinearly parametrized solution fields that conserve given quantities at each time step. We present numerical experiments that show the effectiveness of our proposed method to preserve given quantities up to machine precision. The talk is based on our preprint [1]. [1] P. Schwerdtner, P. Schulze, J. Berman, and B. Peherstorfer, Nonlinear embeddings for conserving Hamiltonians and other quantities with Neural Galerkin schemes, https://arxiv.org/abs/2310.07485, 2023