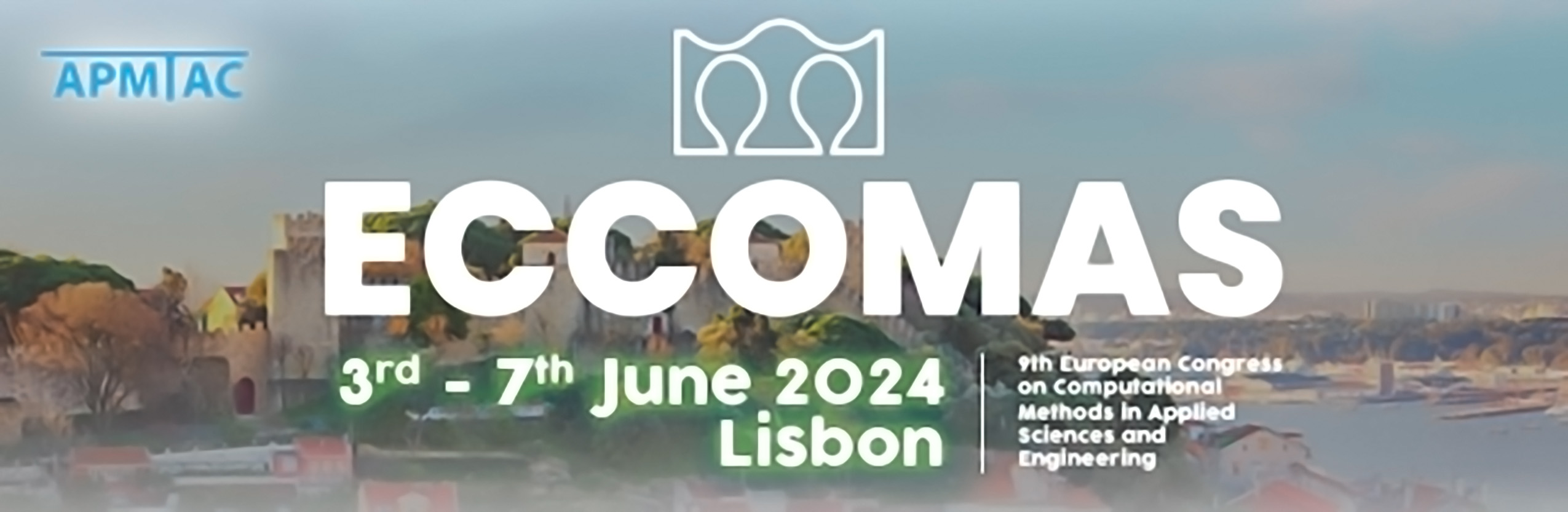
A Phi-FEM approach to train a FNO for variable geometries
Please login to view abstract download link
$\phi$-FEM is a new finite element method, proposed to solve partial differential equations on complex domains, using non-conforming meshes. The method relies on the use of a level-set function $\phi$, which defines the domain and its boundary. In this presentation, we propose a way to combine this method and machine learning techniques (here the Fourier Neural Operator). This method, called $\phi$-FEM-FNO, has been introduced to achieve the resolution of multiple physics problems with good accuracy in real time. For that, the neural operator is trained using synthetic $\phi$-FEM data and the functions defining the governing equations to solve. The preliminary numerical results are very encouraging since for two test cases solving the Poisson-Dirichlet equation on different types of shapes, our approach reaches the precision of a standard finite element method, 100 times faster.