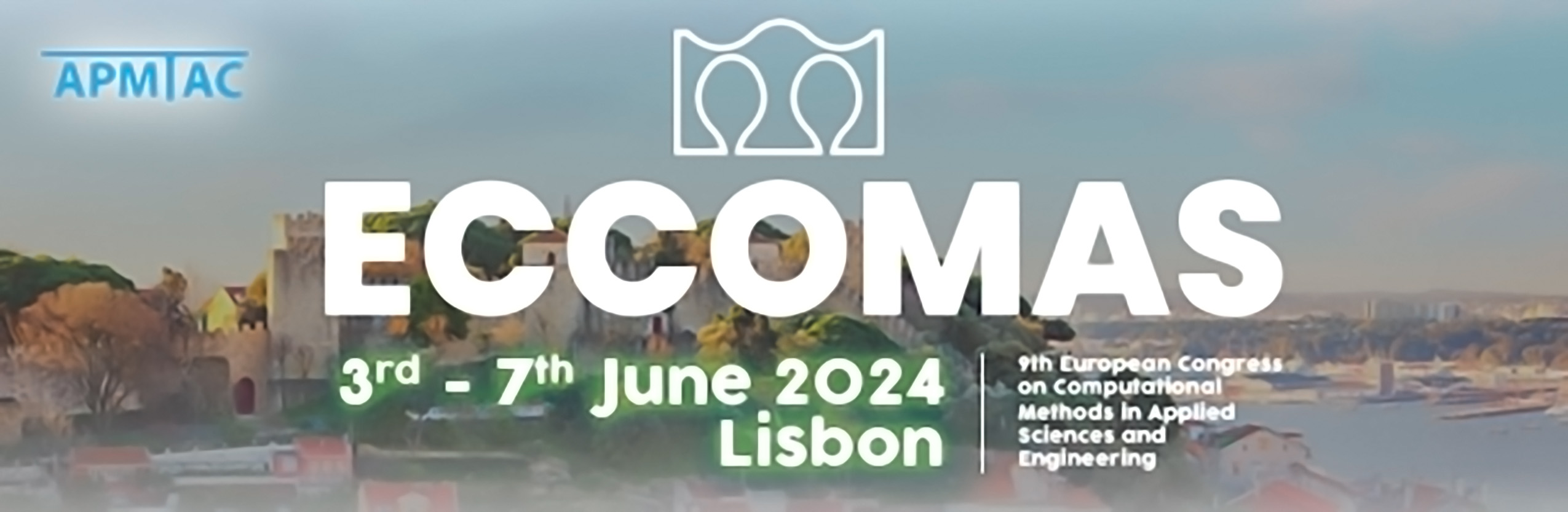
Inverse Problems for Shallow-Water Equations solved by Physics-Informed Machine Learning methods
Please login to view abstract download link
Faced with the socio-economic challenges of flood forecasting, in a context of climate change, multi-scale modeling approaches that take advantage of the maximum amount of information available are needed to enable accurate and rapid flood forecasts. In this context, the objective of this work is to develop a Physics-Informed Machine Learning method to efficiently perform flood models calibration, which is crucial to ensure accurate forecasts. More precisely, an approach aiming at inferring a spatially-distributed friction coefficient (Manning-Strickler coefficient) from data with a Physics-Informed Neural Network (idea introduced by Lagaris et al. and further developed by Raissi et al.) is proposed. The method consists in considering for the loss function a physical model term corresponding to the 2D Shallow-Water Equations residual and a data discrepancy term. Data are generated with the reference software DassFlow and refer to observations of the free surface height and mass flow rate at various locations and times in the computational domain. The PINN parameters and the spatially-distributed friction parameter are then optimized so that the weighted sum of the physical residual term and the data discrepancy term are minimized. To tackle multi-scale issues, a multiresolution strategy is employed on the friction parameter, thus allowing to initialize the optimization with a coarse friction resolution and refining it iteratively throughout the training for regularization purposes. This work is aimed to be published in Boulenc et al. To illustrate the efficiency of the method and its sensitivity to the friction parameter dimension, two river hydraulic modeling test cases will be discussed. Overall, the proposed method appears efficient and robust. Moreover, it is simple to implement (non-intrusive) compared to more traditional Variational Data Assimilation approaches (Monnier), making it a viable strategy to further enhance for rapid flood forecasts.