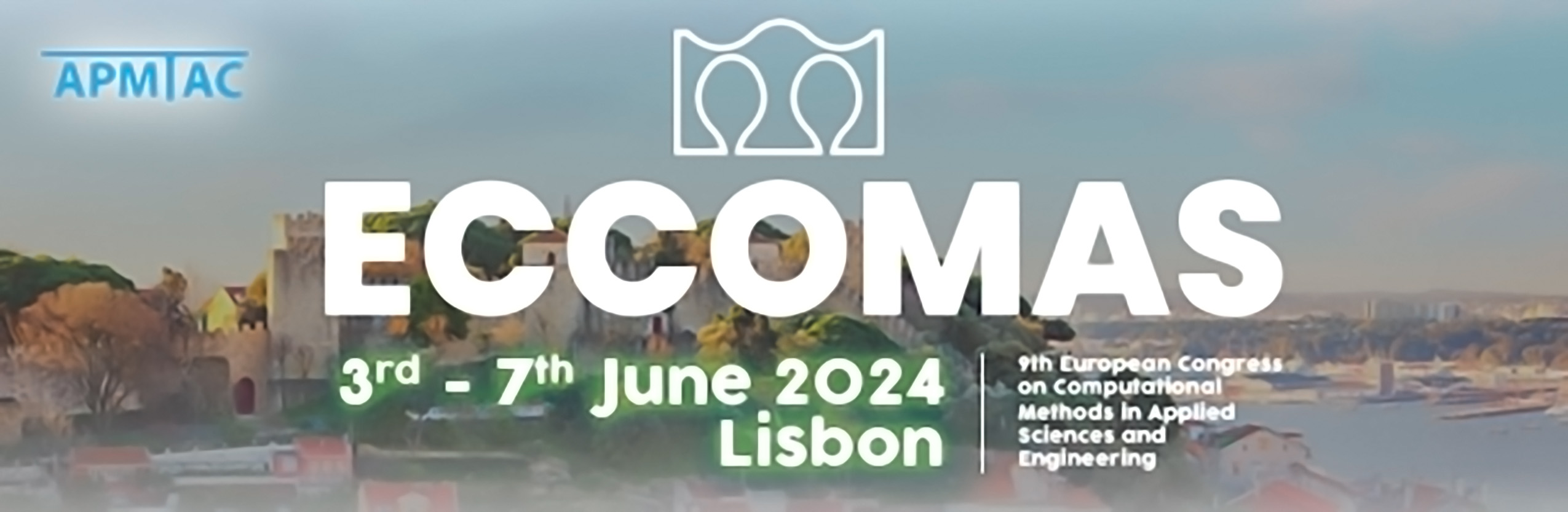
Learning reduced-order models with Bayesian inference and Gaussian processes
Please login to view abstract download link
Credible real-time simulation is a critical enabling factor for digital twin technology, and data-driven model reduction is a natural choice for achieving it. In this talk, we will discuss a probabilistic strategy for the learning of reduced-order representations of high-dimensional dynamical systems, with which a significantly reduced dimensionality guarantees improved efficiency, and the endowed uncertainty quantification certifies computational reliability. The strategy is based on Bayesian reduced-order operator inference, a data-driven method that inherits the formulation structure of projection-based reduced-state governing equations yet without requiring access to full-order solvers. The reduced-order operators are estimated using Bayesian inference with Gaussian priors, and two fundamentally different strategies of likelihood definition will be discussed -- one formulated as linear regression, and the other through Gaussian processes. In particular, the latter aims to improve the learned reduced-order models’ predictive performance when training data are noisy and/or scarce. Given by posterior Gaussian distributions conditioning on solution data, the reduced-order operators probabilistically define a low-dimensional dynamical system for the predominant latent states, and provide an inherently embedded Tikhonov regularization together with a quantification of modeling uncertainties.