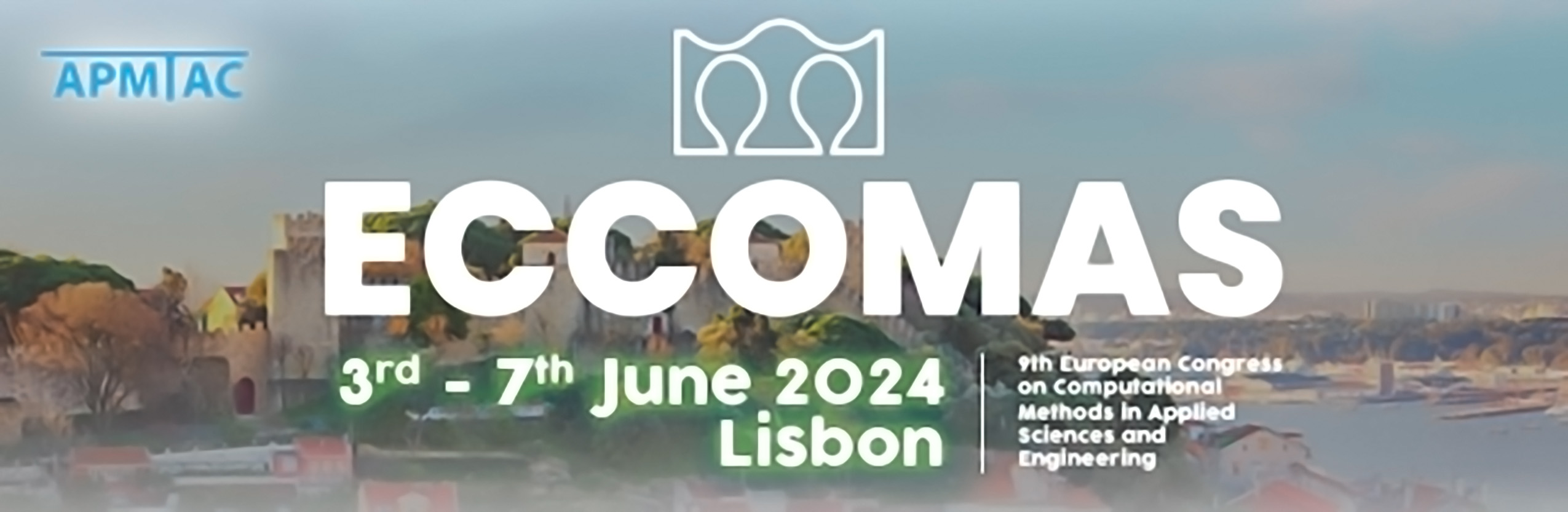
Application of physics-informed deep operator networks to solving unsteady flow problems
Please login to view abstract download link
Physics-informed neural networks (PINNs) \cite{Raissi2019} are a novel class of neural networks characterized by their ability to incorporate physical information (governing equations and their initial and boundary conditions) into the training process. Moreover, PINNs can be trained even only with physical information, conversely without any observation data. Therefore, PINNs have attracted considerable attention as an alternative to numerical solution methods for differential equations, and such trained PINNs are called data-free PINNs (DF-PINNs). However, the validity of DF-PINNs for simulating unsteady flow has not yet been established. %Indeed, it was observed by Chuang and Barba that their DF-PINN failed to reproduce vortex shedding in the simulation of unsteady ow over a cylinder \cite{Chuang2023}. Indeed, it was observed in our recent work that DF-PINNs can reproduce vortex shedding in the simulation of unsteady flow over a cylinder only for a short time duration and only when a sufficient number of collocation points are used in the training. This indicates a limitation of DF-PINNs for long-term simulation of unsteady flow. In this study, to realize long-term simulation of unsteady flow using DF-PINNs, deep operator network (DeepONet) \cite{Lu2019} is introduced, and its validity is discussed. We present the details of our approach and the results of some preliminary numerical tests for canonical problems, such as the advection-diffusion problem.