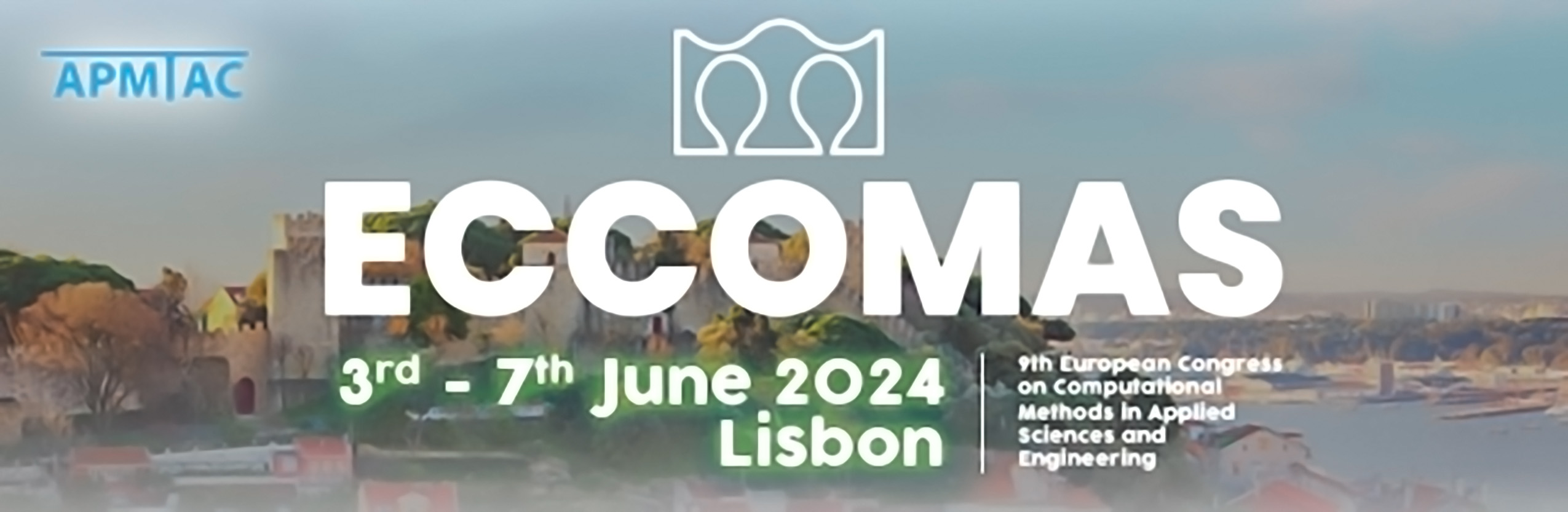
Multiscale Optimization for Programming Material Behavior
Please login to view abstract download link
Programmable metamaterials (PMs) belong to the class of metamaterials offering controllable and variable material behavior. Like metamaterials, they are architectured, cellular materials and exhibit exotic behavior, but are more specifically tailored for engineering purposes. In particular, one objective is to replace complex systems, which capture environmental stimulus through sensors and actors, with only one material, ”programmed” to behave identically, but more robust. Typically, architectured metamaterials consist of unit cells, their basic elemental struc- tures, and usually exhibit homogeneous layouts on micro- or mesoscale. In contrast, PMs reveal heterogeneous layouts of unit cells on same scale, where the variety is related to the parametric design of the cells. By tuning them, the purpose of these parameters is to effectively achieve mechanical target properties on macroscale. We arrive at an inverse design problem for which we propose an adjoint-based optimization framework, similar to topology or material optimization. Beside the construction of cells by humans, we utilize topology optimization for a generative design through machine learning. Overall, the novelty of this framework is that inverse design problems can be solved with a variety of unit cell design options, even for multi-material and multi-physical scenarios. Our work is based on a multiscale approach and data-driven, surrogate modeling technique, allowing a broad range of applications with different classes of unit cells and target functions under finite strain. We begin at an offline phase, where we compute strain-stress responses for all important design and loading cases by using numerical homogenization. For that phase, we allow both explicit, user-defined parametrizations and implicit mapping between designs and mechanical properties of unit cells. Both representations, however, require large data sets in high dimensional spaces. To handle these, we apply model reduction and neural networks in order to compress and cluster the amount of data. A consecutive interpolation yields fast computable surrogate material models, such that fully resolved geometry models can be avoided in the online optimization. In this contribution, we present the complete process chain from unit cells till the final model of the PM, ready to be manufactured. In the end, we show numerical results with different unit cells and compare them to fully resolved simulations and manufactured samples.