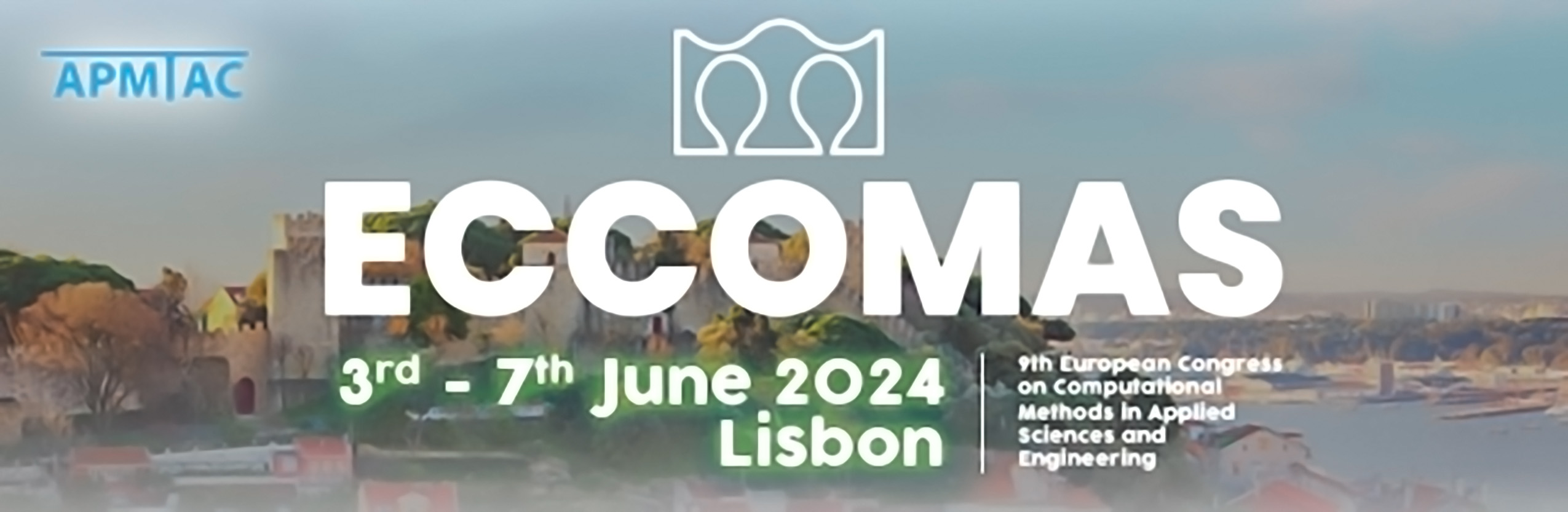
Reduced order models for ICE intake ports design
Please login to view abstract download link
Advancing towards net-zero emissions in the transportation sector is widely recognized as a critical global objective. Internal combustion engines (ICE), potentially powered by renewable fuels, stand as significant assets when appropriately developed to meet this challenging goal. In pursuit of this target, the intricacies of ICE design and development are escalating to fulfill rising expectations for efficiency and emissions control, necessitating the exploration of optimal values for a broad range of parameters. This article concentrates on the design of intake ports for an ICE engine, emphasizing the critical role of Computational Fluid Dynamics (CFD) analysis in supporting the integration of this component into the combustion system with both time and cost effectiveness. To facilitate extensive exploration of the design variable space from early project stages and provide designers with a numerical tool for effectively screening promising design solutions, we develop a non-intrusive Reduced-Order Model (ROM) based on Proper-Orthogonal Decomposition (POD). Using a dataset of solution snapshots corresponding to various geometries and valve lifts, we encode the solution space through POD and employ a regression model to predict the coefficients of the POD expansion for out-of-sample configurations. This enables the reconstruction of the flow field over specific sections and, consequently, the derivation of integral quantities of interest. To train the ROM model, an automated process was developed for executing over a thousand CFD simulations. Improvements to the underlying CFD model have significantly reduced simulation time without compromising accuracy. The effectiveness and drawbacks of this approach are highlighted by studying a parametric intake port problem representative of a real-life industrial application. We compare the performance of different techniques for POD coefficient prediction, including Radial Basis Function (RBF) interpolation, Gaussian Processes (GP), and feed-forward Neural Networks (NN). Additionally, we discuss the advantages and disadvantages of the proposed ROM in comparison to classic response surface methods and machine learning surrogate models trained from integral quantities.