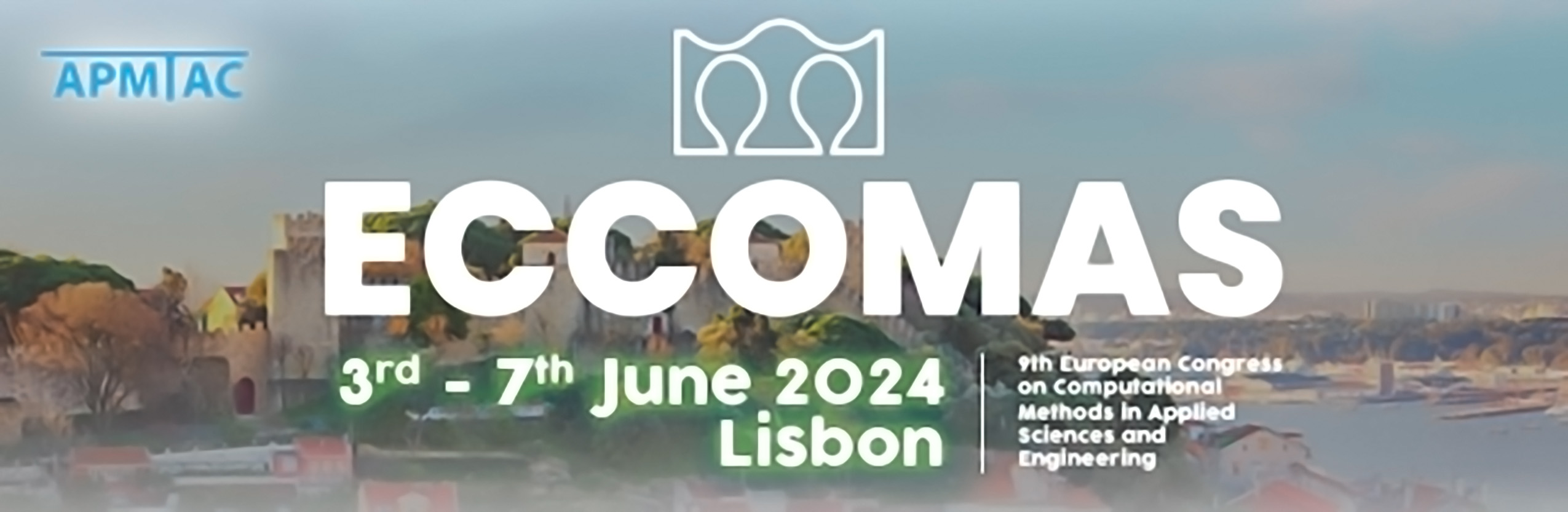
Automated Graph Coarsening: Shell Buckling
Please login to view abstract download link
Predicting buckling loads in spherical shells is essential in a wide range of applications, from industrial tanks and pressure vessels to critical aerospace engineering components. Buckling is highly sensitive to defects or geometric variability, as characterized by the knockdown factor (reduction in buckling load for imperfect shells). For non-trivial shells this factor is an empirical value – the ratio between the measured buckling load and the prediction for a perfect shell [1]. However, the testing procedure is usually destructive and unsuitable for practical applications. Circumventing the experimental route became possible with the advent of finite element analyses, as these simulations can reliably predict the knockdown factor when the geometry is known in detail. Nevertheless, obtaining predictions for practical shells with multiple defects still involves significant computational resources [2]. We created Graph Neural Network (GNN) surrogate models to address this challenge by leveraging GNNs’ ability to predict the buckling response efficiently. We explore the benefits of GNNs in reducing mesh dependency and handling arbitrary and complex domain shapes. We also characterize GNNs’ limitations, particularly in dealing with large node numbers, proposing a practical strategy that properly balances between mesh refinement and the integration of far-field phenomena [3]. We demonstrate the advantages of the proposed strategy in the context of buckling of spherical shells, but we believe that the methodology is applicable in different contexts. REFERENCES [1] J. G. Teng, “Buckling of thin shells: Recent advances and trends,” vol. 49, no. 4, pp. 263–274. [2] F. Derveni, W. Gueissaz, D. Yan, and P. M. Reis, “Probabilistic buckling of imperfect hemispherical shells containing a distribution of defects,” Philosophical Transactions of the Royal Society A: Mathematical, Physical and Engineering Sciences, vol. 381, Feb. 2023. [3] C. Liu, Y. Zhan, J. Wu, C. Li, B. Du, W. Hu, T. Liu, and D. Tao, “Graph pooling for graph neural networks: Progress, challenges, and opportunities,” 2023.