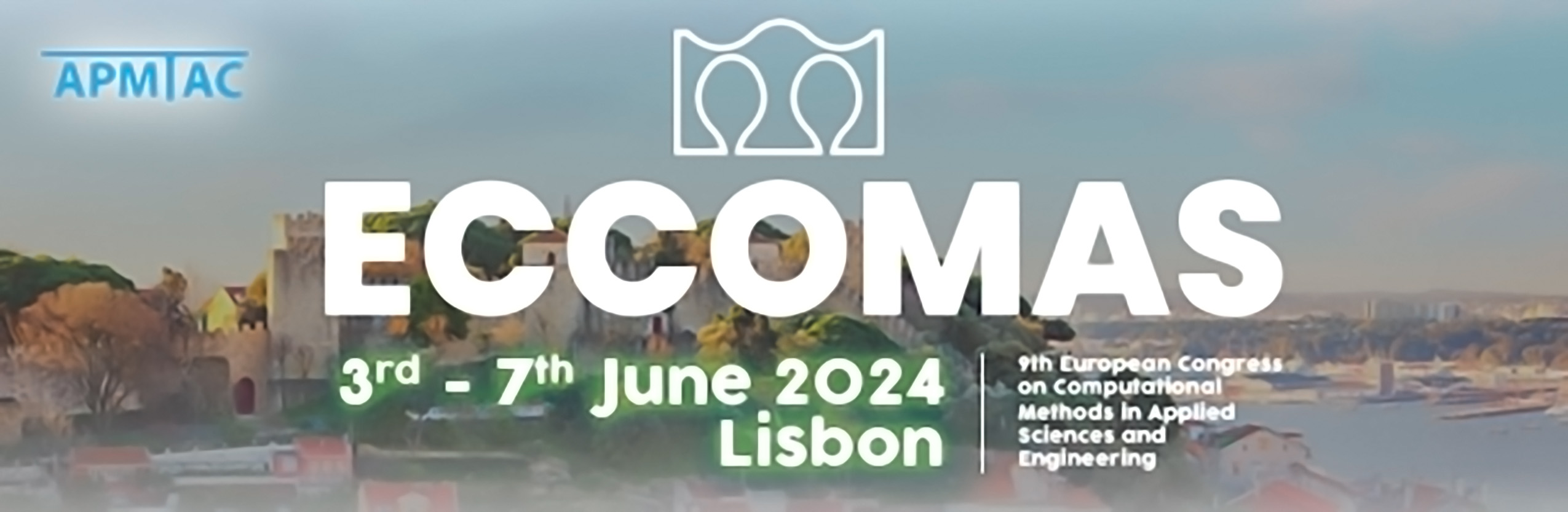
Data-driven parametric reduced order modelling for fast forced vibration response predictions of metamaterial structures via continued fractions
Please login to view abstract download link
The emergence of data-driven reduced order modelling marks a significant advancement in tackling complex industrial problems, primarily due to their ease of application and the increasing availability of scientific data. These models stand out for their nonintrusive nature and capacity to substantially accelerate simulations. By leveraging approximation techniques to predict field variables from sampled data, they circumvent the need for operator projection, potentially leading to faster online calculation than projection based approaches. This is particularly relevant in reduced order modelling of vibro-acoustic metamaterial structures, where the presence of stopband demands large reduced space for accurate dynamic representation, making data-driven approaches increasingly advantageous for efficient online calculations. Although popular approximation techniques like Kriging, polynomial regression, and neural networks are prevalent for interpolating system responses, they fall short in structural dynamics, specifically in the approximation of frequency response functions (FRFs). Their limitations in effectively addressing the poles in FRFs highlights the need for rational functions that align well with the analytical expression of FRFs. In this study, we adapt Thiele's interpolation technique to data-driven parametric model order reduction for forced vibration response analysis of metamaterial structures. The method is compared to several other existing methods for rational approximation such as AAA, vector fitting, Loewner matrix approach.